« Classification naïve bayésienne multinomiale » : différence entre les versions
(Page créée avec « ==en construction== == Définition == XXXXXXXXX == Français == ''' XXXXXXXXX ''' == Anglais == ''' Multinomial naïve Bayes''' With a multinomial event model, samples... ») |
m (Remplacement de texte : « ↵↵==Sources== » par « ==Sources== ») |
||
(7 versions intermédiaires par 2 utilisateurs non affichées) | |||
Ligne 1 : | Ligne 1 : | ||
== | == Définition == | ||
[[Classification naïve bayésienne]] où les attributs suivent une [[distribution multinomiale]]. | |||
== | ==Compléments== | ||
On emploie parfois ''polynomiale'' à la place de ''multinomiale'' et ''bayésienne naïve'' plutôt que ''naïve bayésienne''. | |||
== Français == | == Français == | ||
''' | ''' classification naïve bayésienne multinomiale ''' | ||
''' classification naïve de Bayes multinomiale ''' | |||
''' classification naïve bayésienne polynomiale ''' | |||
''' classification naïve de Bayse polynomiale ''' | |||
== Anglais == | == Anglais == | ||
''' | ''' multinomial naïve Bayes classification''' | ||
With a multinomial event model, samples (feature vectors) represent the frequencies with which certain events have been generated by a multinomial {\displaystyle (p_{1},\dots ,p_{n})}(p_1, \dots, p_n) where {\displaystyle p_{i}}p_{i} is the probability that event i occurs (or K such multinomials in the multiclass case). A feature vector {\displaystyle \mathbf {x} =(x_{1},\dots ,x_{n})}{\mathbf {x}}=(x_{1},\dots ,x_{n}) is then a histogram, with {\displaystyle x_{i}}x_{i} counting the number of times event i was observed in a particular instance. This is the event model typically used for document classification, with events representing the occurrence of a word in a single document (see bag of words assumption). The likelihood of observing a histogram x is given by | <!-- With a multinomial event model, samples (feature vectors) represent the frequencies with which certain events have been generated by a multinomial {\displaystyle (p_{1},\dots ,p_{n})}(p_1, \dots, p_n) where {\displaystyle p_{i}}p_{i} is the probability that event i occurs (or K such multinomials in the multiclass case). A feature vector {\displaystyle \mathbf {x} =(x_{1},\dots ,x_{n})}{\mathbf {x}}=(x_{1},\dots ,x_{n}) is then a histogram, with {\displaystyle x_{i}}x_{i} counting the number of times event i was observed in a particular instance. This is the event model typically used for document classification, with events representing the occurrence of a word in a single document (see bag of words assumption). The likelihood of observing a histogram x is given by | ||
--> | |||
==Sources== | |||
[https://en.wikipedia.org/wiki/Naive_Bayes_classifier#Multinomial_na%C3%AFve_Bayes Source : Wikipedia Machine Learning ] | [https://en.wikipedia.org/wiki/Naive_Bayes_classifier#Multinomial_na%C3%AFve_Bayes Source : Wikipedia Machine Learning ] | ||
[[Catégorie:GRAND LEXIQUE FRANÇAIS]] | |||
[[Catégorie: | |||
Dernière version du 30 août 2024 à 17:58
Définition
Classification naïve bayésienne où les attributs suivent une distribution multinomiale.
Compléments
On emploie parfois polynomiale à la place de multinomiale et bayésienne naïve plutôt que naïve bayésienne.
Français
classification naïve bayésienne multinomiale
classification naïve de Bayes multinomiale
classification naïve bayésienne polynomiale
classification naïve de Bayse polynomiale
Anglais
multinomial naïve Bayes classification
Sources
Contributeurs: Patrick Drouin, wiki
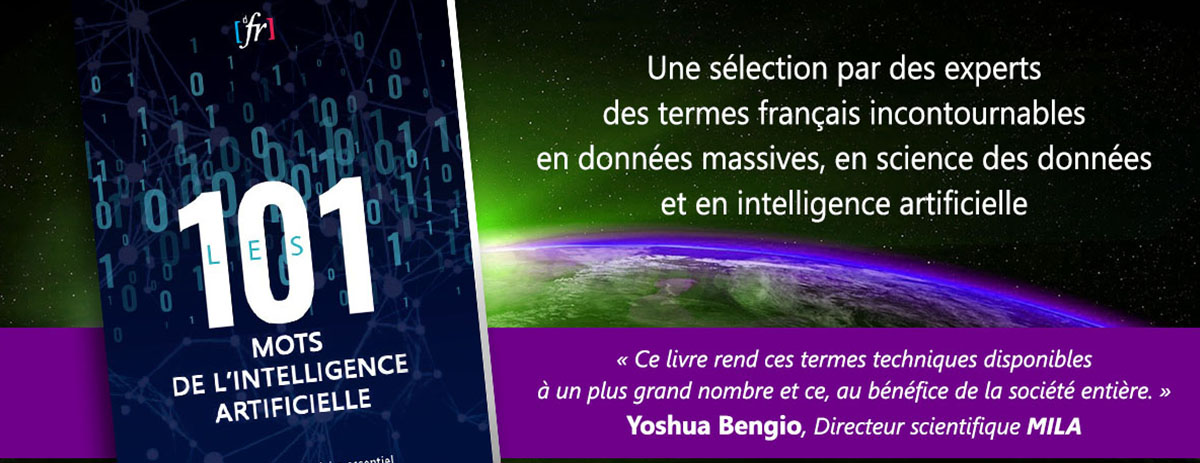