« Algorithme évolutionniste » : différence entre les versions
m (Remplacement de texte — « Termes privilégiés » par « Français ») |
(Ajustement de quelques sections de la fiche.) Balise : Éditeur de wikicode 2017 |
||
Ligne 1 : | Ligne 1 : | ||
== Domaine == | == Domaine == | ||
[[Category: | [[Category:Vocabulaire]]<br/> | ||
[[Category:Intelligence artificielle]]Intelligence artificielle<br/> | |||
[[Category:Apprentissage automatique]] Apprentissage automatique<br/> | |||
[[Category:Apprentissage profond]] Apprentissage profond<br/> | |||
[[Category:Termino 2019]] | |||
[[Category:Scotty]] | |||
== Définition == | == Définition == | ||
Famille d’algorithmes stochastiques qui s'inspirent du processus de sélection naturelle darwinien dans lequel les membres les moins adaptés de la population sont éliminés, tandis que les membres adaptés sont autorisés à survivre et à continuer jusqu'à ce que de meilleures solutions soient déterminées. | |||
Notes | |||
Ces algorithmes sont utilisés pour résoudre des problèmes qui prendraient beaucoup trop de temps à être traité de manière exhaustive. Lorsqu'ils sont utilisés seuls, ils sont généralement appliqués à des problèmes combinatoires. Ils sont cependant souvent utilisés en tandem avec un second algorithme en ayant comme fonction de trouver rapidement un point de départ optimisé pour ce dernier. | |||
Ligne 9 : | Ligne 22 : | ||
== Français == | == Français == | ||
'''algorithme évolutionnaire''' | |||
Ligne 15 : | Ligne 28 : | ||
== Anglais == | == Anglais == | ||
'''evolutionary algorithm''' | |||
In artificial intelligence, an evolutionary algorithm (EA) is a subset of evolutionary computation, a generic population-based metaheuristic optimization algorithm. An EA uses mechanisms inspired by biological evolution, such as reproduction, mutation, recombination, and selection. Candidate solutions to the optimization problem play the role of individuals in a population, and the fitness function determines the quality of the solutions (see also loss function). Evolution of the population then takes place after the repeated application of the above operators. | |||
In artificial intelligence, an evolutionary algorithm (EA) is a subset of evolutionary computation | |||
Evolutionary algorithms often perform well approximating solutions to all types of problems because they ideally do not make any assumption about the underlying fitness landscape. Techniques from evolutionary algorithms applied to the modeling of biological evolution are generally limited to explorations of microevolutionary processes and planning models based upon cellular processes. In most real applications of EAs, computational complexity is a prohibiting factor. In fact, this computational complexity is due to fitness function evaluation. Fitness approximation is one of the solutions to overcome this difficulty. However, seemingly simple EA can solve often complex problems; therefore, there may be no direct link between algorithm complexity and problem complexity. | Evolutionary algorithms often perform well approximating solutions to all types of problems because they ideally do not make any assumption about the underlying fitness landscape. Techniques from evolutionary algorithms applied to the modeling of biological evolution are generally limited to explorations of microevolutionary processes and planning models based upon cellular processes. In most real applications of EAs, computational complexity is a prohibiting factor. In fact, this computational complexity is due to fitness function evaluation. Fitness approximation is one of the solutions to overcome this difficulty. However, seemingly simple EA can solve often complex problems; therefore, there may be no direct link between algorithm complexity and problem complexity. |
Version du 3 juin 2019 à 10:36
Domaine
Intelligence artificielle
Apprentissage automatique
Apprentissage profond
Définition
Famille d’algorithmes stochastiques qui s'inspirent du processus de sélection naturelle darwinien dans lequel les membres les moins adaptés de la population sont éliminés, tandis que les membres adaptés sont autorisés à survivre et à continuer jusqu'à ce que de meilleures solutions soient déterminées.
Notes
Ces algorithmes sont utilisés pour résoudre des problèmes qui prendraient beaucoup trop de temps à être traité de manière exhaustive. Lorsqu'ils sont utilisés seuls, ils sont généralement appliqués à des problèmes combinatoires. Ils sont cependant souvent utilisés en tandem avec un second algorithme en ayant comme fonction de trouver rapidement un point de départ optimisé pour ce dernier.
Français
algorithme évolutionnaire
Anglais
evolutionary algorithm
In artificial intelligence, an evolutionary algorithm (EA) is a subset of evolutionary computation, a generic population-based metaheuristic optimization algorithm. An EA uses mechanisms inspired by biological evolution, such as reproduction, mutation, recombination, and selection. Candidate solutions to the optimization problem play the role of individuals in a population, and the fitness function determines the quality of the solutions (see also loss function). Evolution of the population then takes place after the repeated application of the above operators.
Evolutionary algorithms often perform well approximating solutions to all types of problems because they ideally do not make any assumption about the underlying fitness landscape. Techniques from evolutionary algorithms applied to the modeling of biological evolution are generally limited to explorations of microevolutionary processes and planning models based upon cellular processes. In most real applications of EAs, computational complexity is a prohibiting factor. In fact, this computational complexity is due to fitness function evaluation. Fitness approximation is one of the solutions to overcome this difficulty. However, seemingly simple EA can solve often complex problems; therefore, there may be no direct link between algorithm complexity and problem complexity.
Contributeurs: Claude Coulombe, Jacques Barolet, Julie Roy, Patrick Drouin, wiki
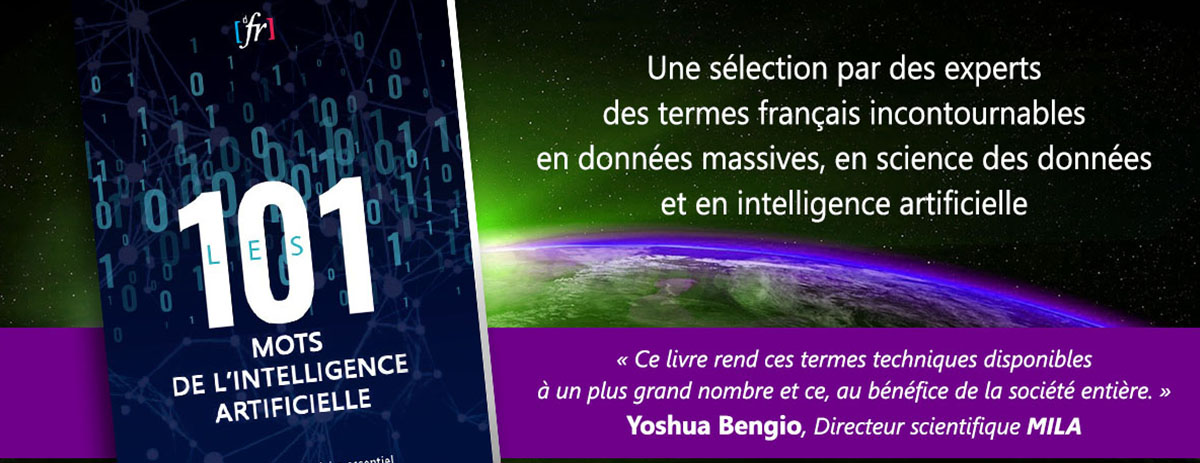