« Extinction de neurone » : différence entre les versions
Aucun résumé des modifications |
Aucun résumé des modifications |
||
Ligne 2 : | Ligne 2 : | ||
[[category:Vocabulary]] Vocabulary<br /> | [[category:Vocabulary]] Vocabulary<br /> | ||
[[Category:Claude]]Claude<br /> | |||
[[Catégorie:Apprentissage profond]] Apprentissage profond | [[Catégorie:Apprentissage profond]] Apprentissage profond | ||
== Définition == | == Définition == | ||
injection de bruit pour rendre le réseau plus robuste, équivalent <br /> | |||
<br /> | |||
== Termes privilégiés == | == Termes privilégiés == | ||
===neurone éteint === | |||
=== trou de réseau === | |||
=== trou de neurone === | |||
=== maille de réseau === | |||
=== défaut de réseau === | |||
=== Point mort === | === Point mort === | ||
<br /> | |||
<br /> | |||
== Anglais == | == Anglais == | ||
===Dropout=== | |||
Dropout is a regularization technique for Neural Networks that prevents overfitting. It prevents neurons from co-adapting by randomly setting a fraction of them to 0 at each training iteration. Dropout can be interpreted in various ways, such as randomly sampling from an exponential number of different networks. Dropout layers first gained popularity through their use in CNNs, but have since been applied to other layers, including input embeddings or recurrent networks. | Dropout is a regularization technique for Neural Networks that prevents overfitting. It prevents neurons from co-adapting by randomly setting a fraction of them to 0 at each training iteration. Dropout can be interpreted in various ways, such as randomly sampling from an exponential number of different networks. Dropout layers first gained popularity through their use in CNNs, but have since been applied to other layers, including input embeddings or recurrent networks. | ||
* Dropout: A Simple Way to Prevent Neural Networks from Overfitting | * Dropout: A Simple Way to Prevent Neural Networks from Overfitting | ||
* Recurrent Neural Network Regularization | * Recurrent Neural Network Regularization | ||
<br /> | |||
<br /> | |||
<br /> | |||
<br /> | |||
<br /> |
Version du 28 mars 2018 à 13:30
Domaine
Vocabulary
Claude
Apprentissage profond
Définition
injection de bruit pour rendre le réseau plus robuste, équivalent
Termes privilégiés
neurone éteint
trou de réseau
trou de neurone
maille de réseau
défaut de réseau
Point mort
Anglais
Dropout
Dropout is a regularization technique for Neural Networks that prevents overfitting. It prevents neurons from co-adapting by randomly setting a fraction of them to 0 at each training iteration. Dropout can be interpreted in various ways, such as randomly sampling from an exponential number of different networks. Dropout layers first gained popularity through their use in CNNs, but have since been applied to other layers, including input embeddings or recurrent networks.
- Dropout: A Simple Way to Prevent Neural Networks from Overfitting
- Recurrent Neural Network Regularization
Contributeurs: Claude Coulombe, Jacques Barolet, Julie Roy, Patrick Drouin, wiki
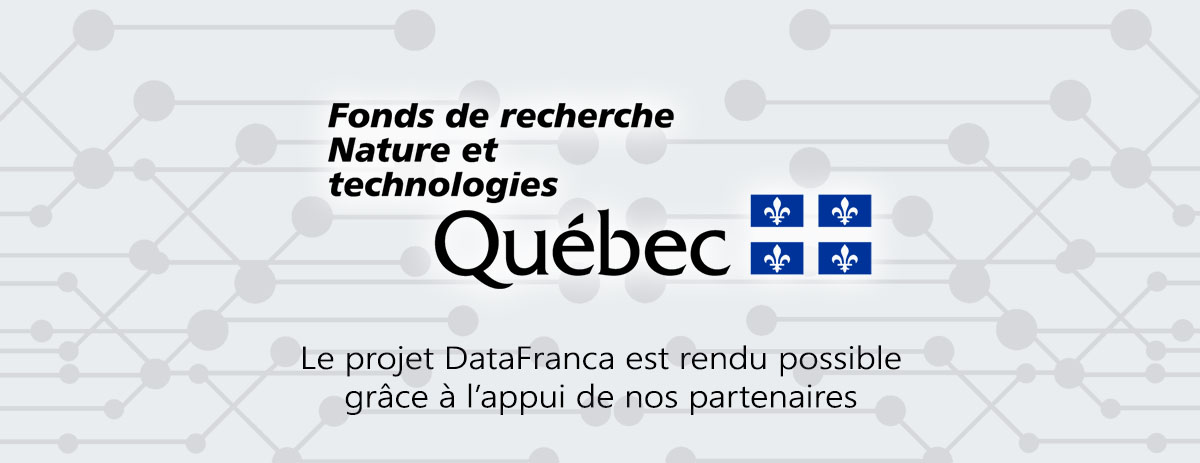