« Carte de diffusion » : différence entre les versions
(Page créée avec « ==en construction== == Définition == XXXXXXXXX == Français == ''' XXXXXXXXX ''' == Anglais == ''' Diffusion map''' Diffusion maps is a dimensionality reduction or fe... ») |
m (ClaireGorjux a déplacé la page Diffusion map vers Carte de diffusion) |
(Aucune différence)
|
Version du 24 octobre 2021 à 16:01
en construction
Définition
XXXXXXXXX
Français
XXXXXXXXX
Anglais
Diffusion map
Diffusion maps is a dimensionality reduction or feature extraction algorithm introduced by Coifman and Lafon[1][2][3][4] which computes a family of embeddings of a data set into Euclidean space (often low-dimensional) whose coordinates can be computed from the eigenvectors and eigenvalues of a diffusion operator on the data. The Euclidean distance between points in the embedded space is equal to the "diffusion distance" between probability distributions centered at those points. Different from linear dimensionality reduction methods such as principal component analysis (PCA) and multi-dimensional scaling (MDS), diffusion maps is part of the family of nonlinear dimensionality reduction methods which focus on discovering the underlying manifold that the data has been sampled from. By integrating local similarities at different scales, diffusion maps give a global description of the data-set. Compared with other methods, the diffusion map algorithm is robust to noise perturbation and computationally inexpensive.
Contributeurs: Claire Gorjux, wiki
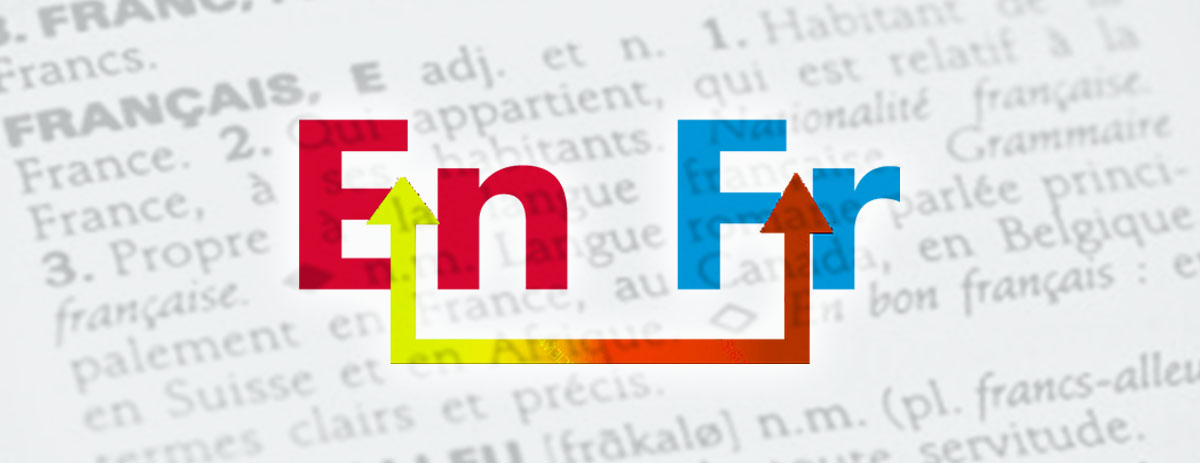