« Amplification de gradient » : différence entre les versions
(Page créée avec « ==en construction== == Définition == XXXXXXXXX == Français == ''' XXXXXXXXX ''' == Anglais == ''' Gradient boosting''' Gradient boosting is a machine learning techni... ») |
m (Imeziani a déplacé la page Gradient boosting vers Amplification de gradient) |
(Aucune différence)
|
Version du 24 novembre 2021 à 14:02
en construction
Définition
XXXXXXXXX
Français
XXXXXXXXX
Anglais
Gradient boosting
Gradient boosting is a machine learning technique for regression and classification problems, which produces a prediction model in the form of an ensemble of weak prediction models, typically decision trees. It builds the model in a stage-wise fashion like other boosting methods do, and it generalizes them by allowing optimization of an arbitrary differentiable loss function.
The idea of gradient boosting originated in the observation by Leo Breiman that boosting can be interpreted as an optimization algorithm on a suitable cost function.[1] Explicit regression gradient boosting algorithms were subsequently developed by Jerome H. Friedman,[2][3] simultaneously with the more general functional gradient boosting perspective of Llew Mason, Jonathan Baxter, Peter Bartlett and Marcus Frean.[4][5] The latter two papers introduced the view of boosting algorithms as iterative functional gradient descent algorithms. That is, algorithms that optimize a cost function over function space by iteratively choosing a function (weak hypothesis) that points in the negative gradient direction. This functional gradient view of boosting has led to the development of boosting algorithms in many areas of machine learning and statistics beyond regression and classification.
Contributeurs: Imane Meziani, wiki
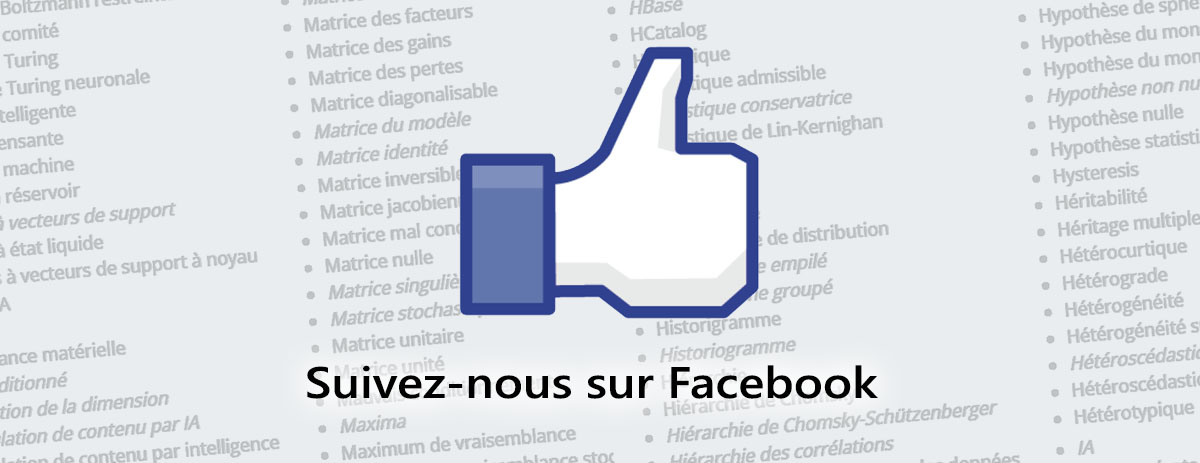