« Séparateur à vaste marge à noyau » : différence entre les versions
(Page créée avec « The kernel support vector machine is essentially the same as the standard SVM, with a cool trick that allows it to discover non-linear decision boundaries. Instead of us... ») |
Aucun résumé des modifications |
||
Ligne 1 : | Ligne 1 : | ||
== Domaine == | |||
[[Category:Vocabulary]]Vocabulary<br /> | |||
<br /> | |||
== Définition == | |||
texte ici | |||
<br /> | |||
== Français == | |||
'''terme_français''' | |||
<br /> | |||
== Anglais == | |||
'''Kernel Support Vector Machines''' | |||
The kernel support vector machine is essentially the same as the standard SVM, with a cool trick that allows it to discover non-linear decision boundaries. | The kernel support vector machine is essentially the same as the standard SVM, with a cool trick that allows it to discover non-linear decision boundaries. | ||
Instead of using the data as-is, we throw our data into something called a kernel. The kernel is any function that takes an input with a given dimensionality and returns an output with higher dimensionality, effectively adding more features to your examples. | Instead of using the data as-is, we throw our data into something called a kernel. The kernel is any function that takes an input with a given dimensionality and returns an output with higher dimensionality, effectively adding more features to your examples. |
Version du 9 mars 2019 à 10:32
Domaine
Vocabulary
Définition
texte ici
Français
terme_français
Anglais
Kernel Support Vector Machines
The kernel support vector machine is essentially the same as the standard SVM, with a cool trick that allows it to discover non-linear decision boundaries.
Instead of using the data as-is, we throw our data into something called a kernel. The kernel is any function that takes an input with a given dimensionality and returns an output with higher dimensionality, effectively adding more features to your examples.
Contributeurs: Claude Coulombe, Gérard Pelletier, Jacques Barolet, wiki
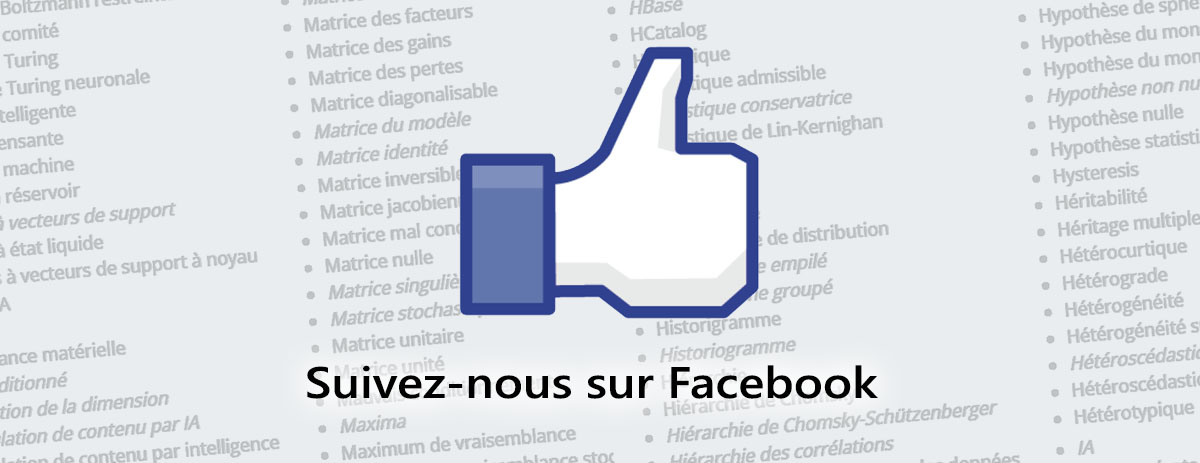