« Apprentissage par renforcement et rétroaction humaine » : différence entre les versions
(Page créée avec « ==en construction== == Définition == XXXXXXXXX == Français == ''' XXXXXXXXX ''' == Anglais == ''' Reinforcement Learning from Human Feedback ''' '''RLHF''' To unde... ») |
Aucun résumé des modifications |
||
Ligne 2 : | Ligne 2 : | ||
== Définition == | == Définition == | ||
En apprentissage automatique, l'apprentissage par renforcement et rétroaction humaine (ARRH) est une technique qui entraîne un modèle de récompense à partir de la rétroaction humaine et utilise le modèle comme fonction de récompense pour optimiser la politique d'un agent à l'aide de l'[[apprentissage par renforcement]] grâce à un algorithme d'optimisation. | |||
== Français == | == Français == | ||
''' | ''' apprentissage par renforcement et rétroaction humaine ''' | ||
'''ARRH''' | |||
== Anglais == | == Anglais == | ||
''' | ''' reinforcement learning from human feedback ''' | ||
'''RLHF''' | '''RLHF''' | ||
''' reinforcement learning from human preferences ''' | |||
<!-- To understand RLHF, we first need to understand the process of training a model like ChatGPT and where RLHF fits in, which is the focus of the first section of this post. The following 3 sections cover the 3 phases of ChatGPT development. For each phase, I’ll discuss the goal for that phase, the intuition for why this phase is needed, and the corresponding mathematical formulation for those who want to see more technical detail. | |||
Currently, RLHF is not yet widely used in the industry except for a few big key players – OpenAI, DeepMind, and Anthropic. However, I’ve seen many work-in-progress efforts using RLHF, so I wouldn’t be surprised to see RLHF used more in the future. | Currently, RLHF is not yet widely used in the industry except for a few big key players – OpenAI, DeepMind, and Anthropic. However, I’ve seen many work-in-progress efforts using RLHF, so I wouldn’t be surprised to see RLHF used more in the future. | ||
--- | --- | ||
Learning from instructions and human feedback are thought to be at the core of recent advances in instruction following large language models (LLMs). While recent efforts such as Open Assistant, Vicuna, and Alpaca have advanced our understanding of instruction fine-tuning, the same cannot be said for RLHF-style algorithms that learn directly from human feedback. AlpacaFarm aims to address this gap by enabling fast, low-cost research and development on methods that learn from human feedback. We identify three main difficulties with studying RLHF-style algorithms: the high cost of human preference data, the lack of trustworthy evaluation, and the absence of reference implementations. | Learning from instructions and human feedback are thought to be at the core of recent advances in instruction following large language models (LLMs). While recent efforts such as Open Assistant, Vicuna, and Alpaca have advanced our understanding of instruction fine-tuning, the same cannot be said for RLHF-style algorithms that learn directly from human feedback. AlpacaFarm aims to address this gap by enabling fast, low-cost research and development on methods that learn from human feedback. We identify three main difficulties with studying RLHF-style algorithms: the high cost of human preference data, the lack of trustworthy evaluation, and the absence of reference implementations. --> | ||
Ligne 25 : | Ligne 32 : | ||
[https://crfm.stanford.edu/2023/05/22/alpaca-farm.html Source : stanford] | [https://crfm.stanford.edu/2023/05/22/alpaca-farm.html Source : stanford] | ||
[https://en.wikipedia.org/wiki/Reinforcement_learning_from_human_feedback Source: Wikipedia] | |||
[[Catégorie:vocabulary]] | [[Catégorie:vocabulary]] |
Version du 29 mai 2023 à 14:11
en construction
Définition
En apprentissage automatique, l'apprentissage par renforcement et rétroaction humaine (ARRH) est une technique qui entraîne un modèle de récompense à partir de la rétroaction humaine et utilise le modèle comme fonction de récompense pour optimiser la politique d'un agent à l'aide de l'apprentissage par renforcement grâce à un algorithme d'optimisation.
Français
apprentissage par renforcement et rétroaction humaine
ARRH
Anglais
reinforcement learning from human feedback
RLHF
reinforcement learning from human preferences
Contributeurs: Arianne , Claude Coulombe, Patrick Drouin, wiki
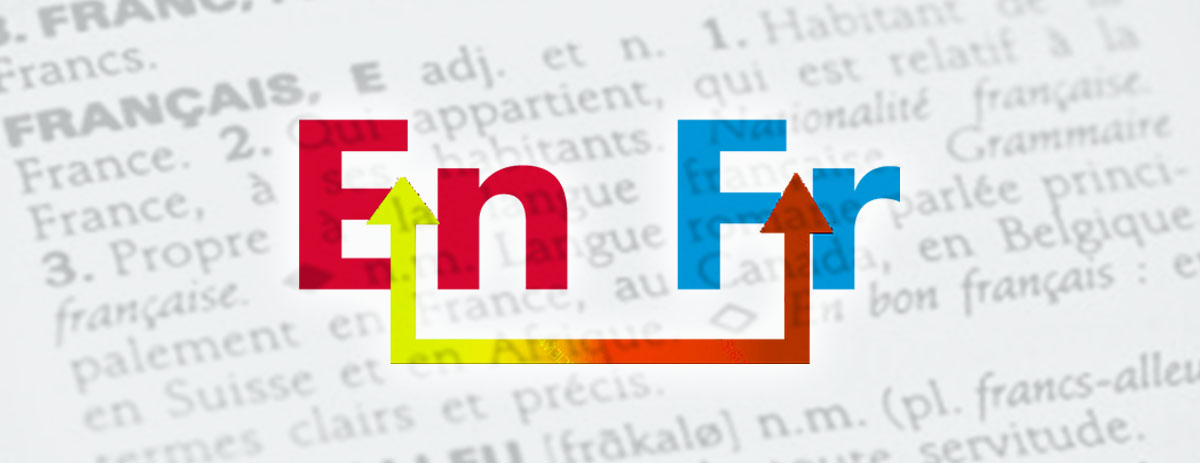