« Raisonnement abstrait » : différence entre les versions
Aucun résumé des modifications |
Aucun résumé des modifications |
||
Ligne 2 : | Ligne 2 : | ||
== Définition == | == Définition == | ||
Le raisonnement abstrait désigne la capacité de résoudre des problèmes complexes en identifiant les régularités et les relations d'un problème à résoudre et en les utilisant pour déduire la solution. Dans un domaine dominé par l'entraînement intensif des modèles avec un grand nombre d'exemples, les modèles de raisonnement abstrait visent à combler l'écart entre l'intelligence fluide humaine et les performances des réseaux neuronaux profonds, qui requièrent un entraînement préalable approfondi. | |||
Voir aussi '''[[Corpus d'Abstraction et de Raisonnement]]''' | Voir aussi '''[[Corpus d'Abstraction et de Raisonnement]]''', '''[[préentraînement]]''' et '''[[réseau de neurones artificiels]]''' | ||
== Compléments == | |||
Le raisonnement abstrait est possible même dans les réseaux neuronaux naïfs (et aléatoires) et ces réseaux peuvent parvenir à ce qui ressemble à un raisonnement abstrait symbolique sans aucun entraînement et, par conséquent, sans aucun rappel de mémoire. | |||
== Français == | == Français == | ||
''' | ''' raisonnement abstrait''' | ||
== Anglais == | == Anglais == | ||
''' | ''' abstract reasoning''' | ||
''' | ''' fluid intelligence''' | ||
''Abstract reasoning is the ability to solve complex problems by identifying regularities and relations in the problem being solved and utilizing them for deducing the solution. Changes in how problems are phrased that seem minor to humans and do not affect their performance can render them unsolvable to networks. In a field dominated by extensive training with an extensive number of examples, abstract reasoning models aim to close the gap between human fluid intelligence and the performance of deep neural networks, which typically require extensive prior training. In fact, abstract reasoning is possible even in naïve and random ANNs and these networks can achieve what looks like symbolic abstract reasoning without any training and, hence, any memory recall.'' | ''Abstract reasoning is the ability to solve complex problems by identifying regularities and relations in the problem being solved and utilizing them for deducing the solution. Changes in how problems are phrased that seem minor to humans and do not affect their performance can render them unsolvable to networks. In a field dominated by extensive training with an extensive number of examples, abstract reasoning models aim to close the gap between human fluid intelligence and the performance of deep neural networks, which typically require extensive prior training. In fact, abstract reasoning is possible even in naïve and random ANNs and these networks can achieve what looks like symbolic abstract reasoning without any training and, hence, any memory recall.'' |
Version du 23 octobre 2024 à 10:38
en construction
Définition
Le raisonnement abstrait désigne la capacité de résoudre des problèmes complexes en identifiant les régularités et les relations d'un problème à résoudre et en les utilisant pour déduire la solution. Dans un domaine dominé par l'entraînement intensif des modèles avec un grand nombre d'exemples, les modèles de raisonnement abstrait visent à combler l'écart entre l'intelligence fluide humaine et les performances des réseaux neuronaux profonds, qui requièrent un entraînement préalable approfondi.
Voir aussi Corpus d'Abstraction et de Raisonnement, préentraînement et réseau de neurones artificiels
Compléments
Le raisonnement abstrait est possible même dans les réseaux neuronaux naïfs (et aléatoires) et ces réseaux peuvent parvenir à ce qui ressemble à un raisonnement abstrait symbolique sans aucun entraînement et, par conséquent, sans aucun rappel de mémoire.
Français
raisonnement abstrait
Anglais
abstract reasoning
fluid intelligence
Abstract reasoning is the ability to solve complex problems by identifying regularities and relations in the problem being solved and utilizing them for deducing the solution. Changes in how problems are phrased that seem minor to humans and do not affect their performance can render them unsolvable to networks. In a field dominated by extensive training with an extensive number of examples, abstract reasoning models aim to close the gap between human fluid intelligence and the performance of deep neural networks, which typically require extensive prior training. In fact, abstract reasoning is possible even in naïve and random ANNs and these networks can achieve what looks like symbolic abstract reasoning without any training and, hence, any memory recall.
Sources
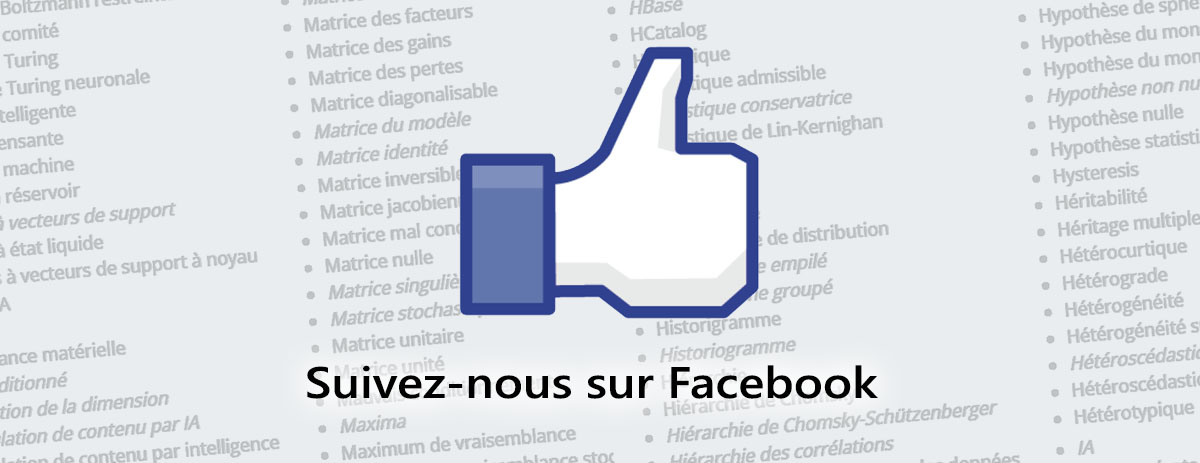