« Expectation Maximization » : différence entre les versions
m (Remplacement de texte — « DeepAI.org ] » par « DeepAI.org ] Catégorie:DeepAI.org ») |
Aucun résumé des modifications |
||
Ligne 1 : | Ligne 1 : | ||
==en construction== | ==en construction== | ||
Voir [[algorithme EM]] | |||
== Définition == | == Définition == |
Version du 13 juillet 2021 à 09:02
en construction
Voir algorithme EM
Définition
XXXXXXXXX
Français
XXXXXXXXX
Anglais
Expectation Maximization
Expectation maximization (EM) is an algorithm that finds the best estimates for model parameters when a dataset is missing information or has hidden latent variables. While this technique can be used to determine the maximum likelihood function, or the “best fit” model for a set of data, EM takes things a step further and works on incomplete data sets. This is achieved by inserting random values for the missing data points, and then estimating a second set of data. The new dataset is used to refine the guesses added to the first, with the process repeating until the algorithm’s termination criterion are met.
Contributeurs: Claire Gorjux, wiki
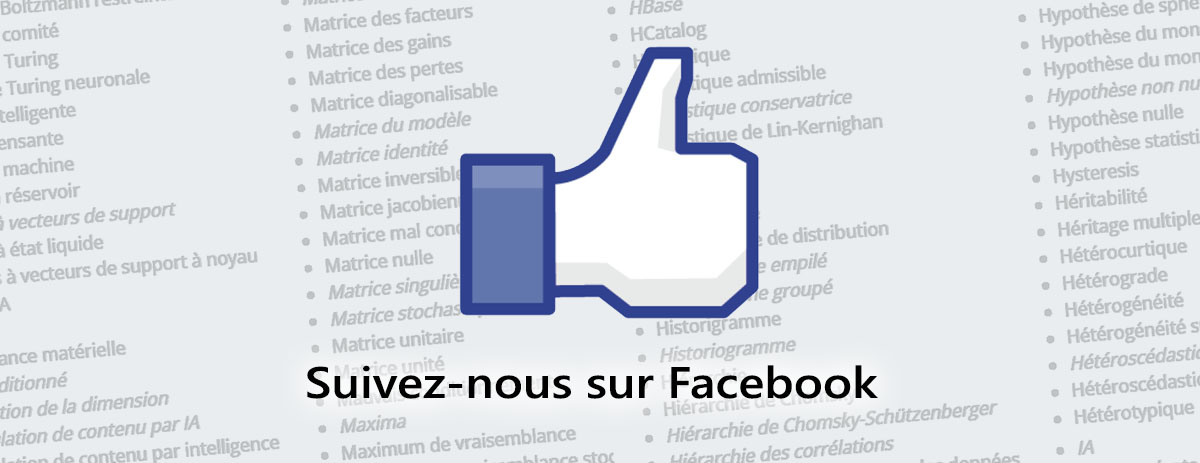