« Borne inférieure variationnelle » : différence entre les versions
Aucun résumé des modifications |
Aucun résumé des modifications |
||
Ligne 1 : | Ligne 1 : | ||
== Définition == | == Définition == | ||
== Français == | == Français == | ||
Ligne 18 : | Ligne 16 : | ||
Noise-contrastive estimation is a sampling loss typically used to train classifiers with a large output vocabulary. Calculating the softmax over a large number of possible classes is prohibitively expensive. Using NCE, we can reduce the problem to binary classification problem by training the classifier to discriminate between samples from the “real” distribution and an artificially generated noise distribution. | Noise-contrastive estimation is a sampling loss typically used to train classifiers with a large output vocabulary. Calculating the softmax over a large number of possible classes is prohibitively expensive. Using NCE, we can reduce the problem to binary classification problem by training the classifier to discriminate between samples from the “real” distribution and an artificially generated noise distribution. | ||
https://deepai.org/machine-learning-glossary-and-terms/noise-contrastive-estimation | [https://deepai.org/machine-learning-glossary-and-terms/noise-contrastive-estimation Source: Deepai] | ||
[[Catégorie:9]] | |||
[[Catégorie:App-profond-livre]] | |||
[[Catégorie:Apprentissage profond]] |
Version du 27 janvier 2021 à 08:52
Définition
Français
Borne inférieure variationnelle
Anglais
Noise contrastive estimation
Source : L'apprentissage profond, Ian Goodfellow, Yoshua Bengio et Aaron Courville Éd. Massot 2018
Noise-contrastive estimation is a sampling loss typically used to train classifiers with a large output vocabulary. Calculating the softmax over a large number of possible classes is prohibitively expensive. Using NCE, we can reduce the problem to binary classification problem by training the classifier to discriminate between samples from the “real” distribution and an artificially generated noise distribution.
Contributeurs: Evan Brach, Imane Meziani, Jean Benoît Morel, Jacques Barolet, wiki
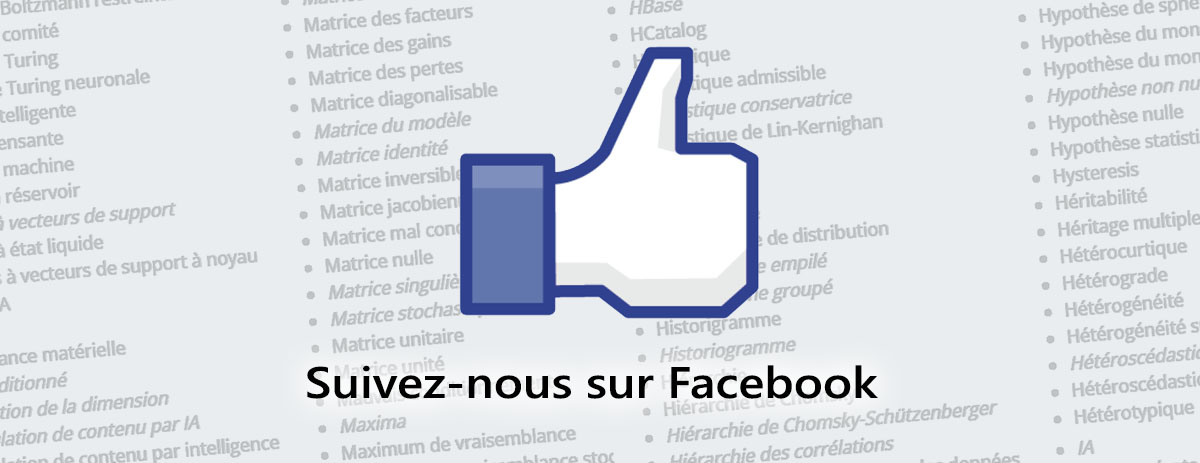