« Méthode d'interprétation agnostique » : différence entre les versions
(Page créée avec « ==en construction== == Définition == XXXXXXXXX == Français == ''' XXXXXXXXX ''' == Anglais == ''' Model-Agnostic Method ''' Interpretable models are models who exp... ») |
Aucun résumé des modifications |
||
Ligne 17 : | Ligne 17 : | ||
[https://towardsdatascience.com/model-agnostic-methods-for-interpreting-any-machine-learning-model-4f10787ef504 | [https://towardsdatascience.com/model-agnostic-methods-for-interpreting-any-machine-learning-model-4f10787ef504 Source : towardsdatascience ] | ||
[[Catégorie:vocabulary]] | [[Catégorie:vocabulary]] |
Version du 20 décembre 2022 à 09:40
en construction
Définition
XXXXXXXXX
Français
XXXXXXXXX
Anglais
Model-Agnostic Method
Interpretable models are models who explain themselves, for instance from a decision tree you can easily extract decision rules. Model-agnostic methods are methods you can use for any machine learning model, from support vector machines to neural networks. It’s not possible to directly interpret most machine learning models. For popular models like random forests, gradient boosted machines and neural networks you need model-agnostic methods. At the moment there are some interesting methods available, like permutation feature importance³, Partial Dependence Plots (PDPs), Individual Conditional Expectation (ICE) plots, global surrogate models, Local Interpretable Model-agnostic Explanations (LIME) and Shapley Additive Explanations (SHAP).
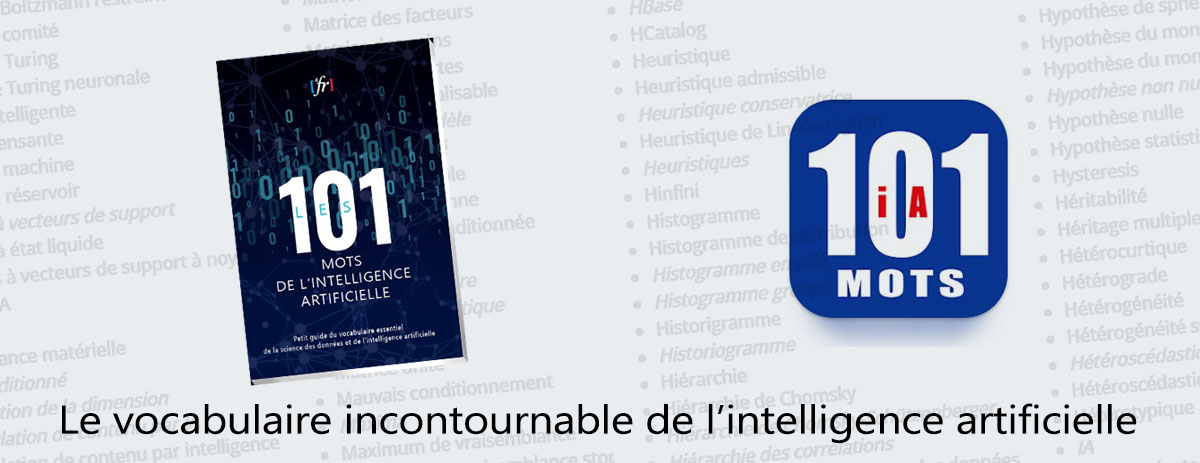
Contributeurs: Imane Meziani, wiki