« Recherche de règles d'association » : différence entre les versions
(Page créée avec « discovering rules for observing A given B. This is closely related to clustering in that we’re attempting to find connections between events. However, the difference is... ») |
Aucun résumé des modifications Balise : Éditeur de wikicode 2017 |
||
Ligne 1 : | Ligne 1 : | ||
== en construction == | |||
[[Catégorie:Intelligence artificielle]] | |||
[[Catégorie:vocabulary]] | |||
== Définition == | |||
== Français == | |||
'''XXXXXXXXXXXXXXX ''' | |||
== Anglais == | |||
'''XXXXXXXXXXXXXXX ''' | |||
discovering rules for observing A given B. This is closely related to clustering in that we’re attempting to find connections between events. However, the difference is the approach. Instead of drawing bounds on a region and seeing if someone fits in that bucket, we use the frequency of a collection of discrete observations to create priors: what’s the probability of observing A, or B, or C? From these, we figure out what the probability is of observing A given B, or observing C given A and B. This is just Bayesian statistics: P(A), P(B | A), P(C | A, B), and so forth. | discovering rules for observing A given B. This is closely related to clustering in that we’re attempting to find connections between events. However, the difference is the approach. Instead of drawing bounds on a region and seeing if someone fits in that bucket, we use the frequency of a collection of discrete observations to create priors: what’s the probability of observing A, or B, or C? From these, we figure out what the probability is of observing A given B, or observing C given A and B. This is just Bayesian statistics: P(A), P(B | A), P(C | A, B), and so forth. | ||
<small> | |||
Machine Learning for Beginners – a How-to Guide | Machine Learning for Beginners – a How-to Guide | ||
https://opendatascience.com/machine-learning-for-beginners/ | https://opendatascience.com/machine-learning-for-beginners/ |
Version du 16 août 2019 à 21:48
en construction
Définition
Français
XXXXXXXXXXXXXXX
Anglais
XXXXXXXXXXXXXXX
discovering rules for observing A given B. This is closely related to clustering in that we’re attempting to find connections between events. However, the difference is the approach. Instead of drawing bounds on a region and seeing if someone fits in that bucket, we use the frequency of a collection of discrete observations to create priors: what’s the probability of observing A, or B, or C? From these, we figure out what the probability is of observing A given B, or observing C given A and B. This is just Bayesian statistics: P(A), P(B | A), P(C | A, B), and so forth.
Machine Learning for Beginners – a How-to Guide https://opendatascience.com/machine-learning-for-beginners/
Contributeurs: Gérard Pelletier, Imane Meziani, Jean Benoît Morel, wiki
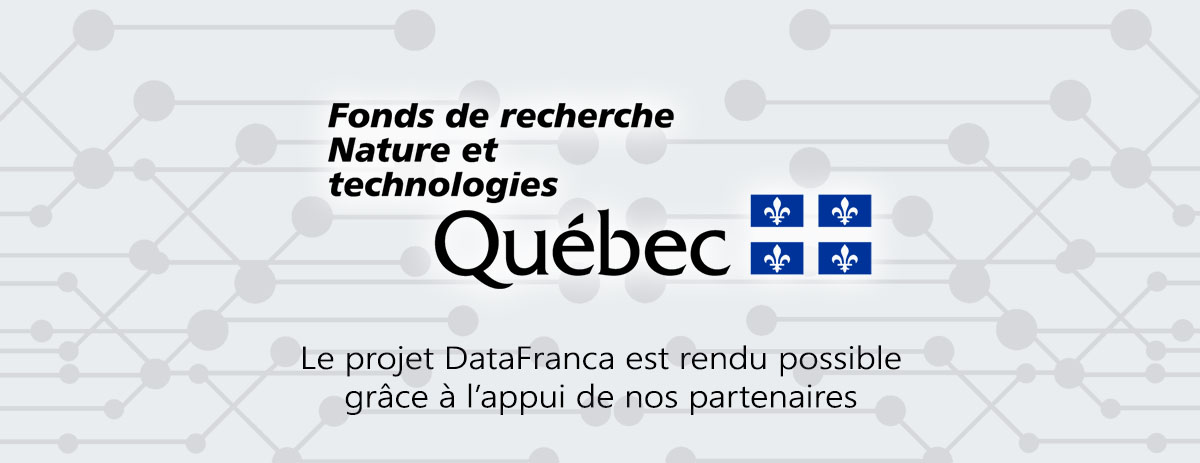