« Descente de gradient stochastique asynchrone » : différence entre les versions
m (Arianne a déplacé la page Asynchronous Stochastic Gradient Descent vers Descente de gradient stochastique asynchrone) |
Aucun résumé des modifications |
||
Ligne 1 : | Ligne 1 : | ||
== Définition == | == Définition == | ||
La descente de gradient stochastique asynchrone est une approche qui vise à améliorer l'efficacité de la formation des réseaux profonds à grande échelle en l'accélérant dans un environnement informatique distribué. Elle permet d'atteindre une vitesse plus rapide puisqu'aucun travailleur n'a besoin d'attendre après les autres et ils ajoutent directement le gradient local au modèle global. | La descente de gradient stochastique asynchrone est une approche qui vise à améliorer l'efficacité de la formation des réseaux profonds à grande échelle en l'accélérant dans un environnement informatique distribué. Elle permet d'atteindre une vitesse plus rapide puisqu'aucun travailleur n'a besoin d'attendre après les autres et ils ajoutent directement le gradient local au modèle global. | ||
Ligne 11 : | Ligne 9 : | ||
== Français == | == Français == | ||
''' | ''' descente de gradient stochastique asynchrone''' | ||
== Anglais == | == Anglais == | ||
''' | ''' asynchronous stochastic gradient descent''' | ||
''' | ''' asynchronous SGD''' | ||
''' ASGD''' | ''' ASGD''' | ||
''Asynchronous | ''Asynchronous stochastic gradient descent (ASGD) is an approach that aims to improve training efficiency of large-scale deep network by accelerating it in a distributed computing environment. It can achieve faster speed due to the fact that no worker need to wait for other and these workers directly add the local gradient to the global model.'' | ||
''It is difficult to tune the hyperparameters, to achieve convergence and linear speedup since the stability of the optimization algorithm is strongly influenced by the asynchronous nature of parameter uptade. ASGD also costs more iterations to converge than sequential SGD and cannot reach accuracy parity of sequential SGD especially when the number of workers is large.'' | ''It is difficult to tune the hyperparameters, to achieve convergence and linear speedup since the stability of the optimization algorithm is strongly influenced by the asynchronous nature of parameter uptade. ASGD also costs more iterations to converge than sequential SGD and cannot reach accuracy parity of sequential SGD especially when the number of workers is large.'' | ||
Ligne 30 : | Ligne 28 : | ||
[https://www.ijcai.org/Proceedings/16/Papers/335.pdf Source : IJCAI-16] | [https://www.ijcai.org/Proceedings/16/Papers/335.pdf Source : IJCAI-16] | ||
[[Catégorie: | [[Catégorie:publication]] |
Version du 30 novembre 2024 à 13:06
Définition
La descente de gradient stochastique asynchrone est une approche qui vise à améliorer l'efficacité de la formation des réseaux profonds à grande échelle en l'accélérant dans un environnement informatique distribué. Elle permet d'atteindre une vitesse plus rapide puisqu'aucun travailleur n'a besoin d'attendre après les autres et ils ajoutent directement le gradient local au modèle global.
Voir aussi descente de gradient stochastique et réseau de neurones artificiels
Compléments
Il est cependant difficile d'ajuster les hyperparamètres pour obtenir une convergence et une accélération linéaire, puisque la stabilité de l'algorithme d'optimisation est fortement influencée par la nature asynchrone de l'évolution des paramètres. La descente de gradient stochastique asynchrone coûte également plus d'itérations pour converger que le SGD séquentiel et ne peut pas atteindre la parité de précision du SGD séquentiel, en particulier lorsque le nombre de travailleurs est élevé.
Français
descente de gradient stochastique asynchrone
Anglais
asynchronous stochastic gradient descent
asynchronous SGD
ASGD
Asynchronous stochastic gradient descent (ASGD) is an approach that aims to improve training efficiency of large-scale deep network by accelerating it in a distributed computing environment. It can achieve faster speed due to the fact that no worker need to wait for other and these workers directly add the local gradient to the global model.
It is difficult to tune the hyperparameters, to achieve convergence and linear speedup since the stability of the optimization algorithm is strongly influenced by the asynchronous nature of parameter uptade. ASGD also costs more iterations to converge than sequential SGD and cannot reach accuracy parity of sequential SGD especially when the number of workers is large.
Source
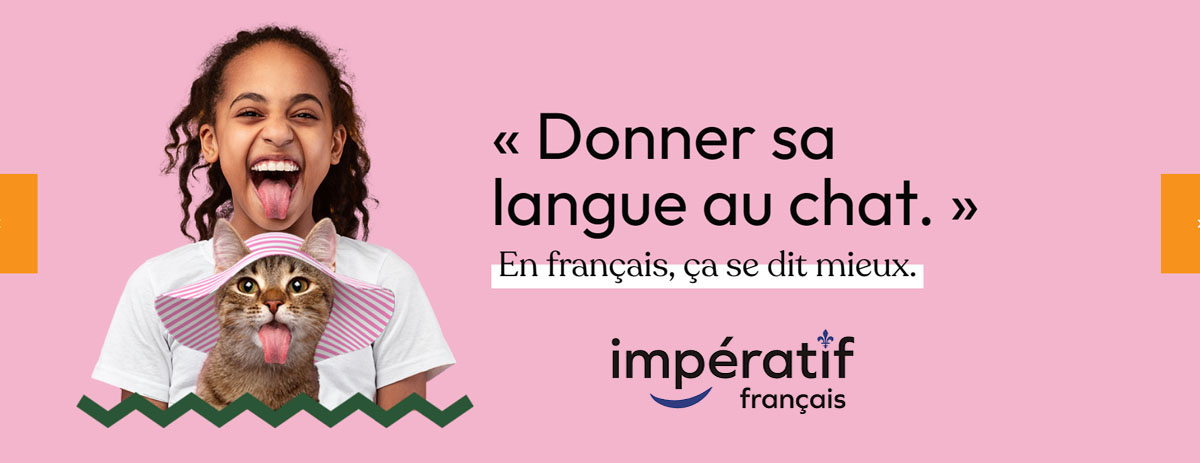