« Machine learning » : différence entre les versions
Aucun résumé des modifications |
Aucun résumé des modifications |
||
Ligne 1 : | Ligne 1 : | ||
== Domaine == | == Domaine == | ||
[[Category:Vocabulary]] | [[Category:Vocabulary]]Vocabulary<br /> | ||
[[Category:Apprentissage profond]] | |||
[[Category:Intelligence artificielle]] | [[Category:Apprentissage profond]]Apprentissage profond<br /> | ||
[[Category:Intelligence artificielle]]Intelligence artificielle<br /> | |||
== Définition == | == Définition == |
Version du 17 mars 2018 à 20:21
Domaine
Vocabulary
Apprentissage profond
Intelligence artificielle
Définition
L'apprentissage automatique (en anglais machine learning, littéralement « l'apprentissage machine ») ou apprentissage statistique.
Champ d'étude de l'intelligence artificielle, concerne la conception, l'analyse, le développement et l'implémentation de méthodes permettant à une machine (au sens large) d'évoluer par un processus systématique, et ainsi de remplir des tâches difficiles ou problématiques par des moyens algorithmiques plus classiques.
Termes privilégiés
<poll> Plusieurs termes sont proposés lequel vous semble le plus juste ? apprentissage machine apprentissage automatique apprentissage artificiel apprentissage statistique </poll>
Anglais
Machine learning
Machine learning is a field of computer science that gives computer systems the ability to "learn" (i.e. progressively improve performance on a specific task) with data, without being explicitly programmed.[1]
The name Machine learning was coined in 1959 by Arthur Samuel.[2] Evolved from the study of pattern recognition and computational learning theory in artificial intelligence,[3] machine learning explores the study and construction of algorithms that can learn from and make predictions on data[4] – such algorithms overcome following strictly static program instructions by making data-driven predictions or decisions,[5]:2 through building a model from sample inputs. Machine learning is employed in a range of computing tasks where designing and programming explicit algorithms with good performance is difficult or infeasible; example applications include email filtering, detection of network intruders or malicious insiders working towards a data breach,[6] optical character recognition (OCR),[7] learning to rank, and computer vision.
Contributeurs: Jacques Barolet, wiki
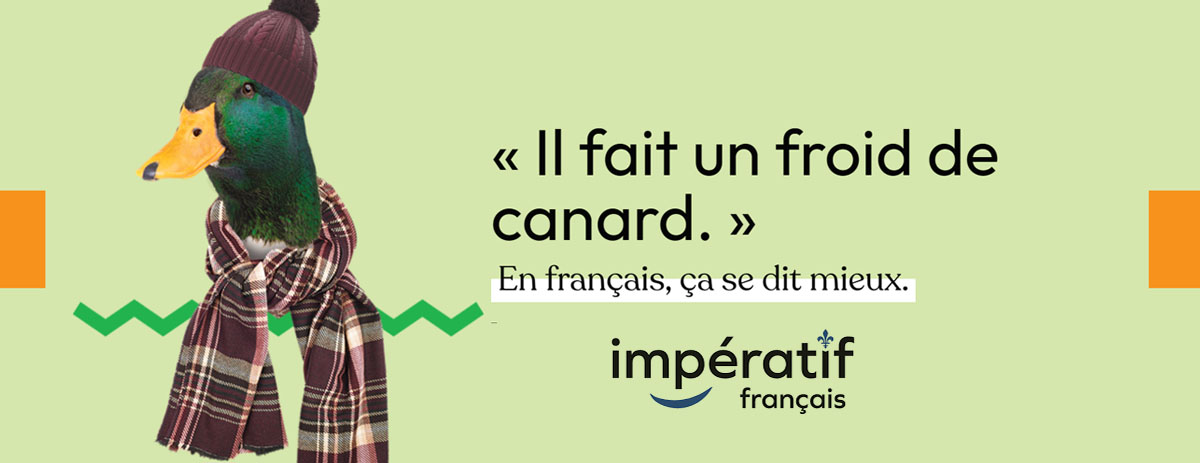