« Partitionnement en k-moyennes » : différence entre les versions
(Page créée avec « ==en construction== == Définition == XXXXXXXXX == Français == ''' XXXXXXXXX ''' == Anglais == ''' k-means clustering''' k-means clustering is a method of vector quan... ») |
Aucun résumé des modifications |
||
Ligne 5 : | Ligne 5 : | ||
== Français == | == Français == | ||
''' | ''' algorithme des centres de groupes''' | ||
== Anglais == | == Anglais == | ||
Ligne 14 : | Ligne 14 : | ||
<small> | <small> | ||
[http://isi.cbs.nl/glossary/term1780.htm Source : ISI ] | |||
[https://en.wikipedia.org/wiki/K-means_clustering Source : Wikipedia Machine Learning ] | [https://en.wikipedia.org/wiki/K-means_clustering Source : Wikipedia Machine Learning ] | ||
Version du 31 janvier 2021 à 16:43
en construction
Définition
XXXXXXXXX
Français
algorithme des centres de groupes
Anglais
k-means clustering
k-means clustering is a method of vector quantization, originally from signal processing, that aims to partition n observations into k clusters in which each observation belongs to the cluster with the nearest mean (cluster centers or cluster centroid), serving as a prototype of the cluster. This results in a partitioning of the data space into Voronoi cells. k-means clustering minimizes within-cluster variances (squared Euclidean distances), but not regular Euclidean distances, which would be the more difficult Weber problem: the mean optimizes squared errors, whereas only the geometric median minimizes Euclidean distances. For instance, better Euclidean solutions can be found using k-medians and k-medoids.
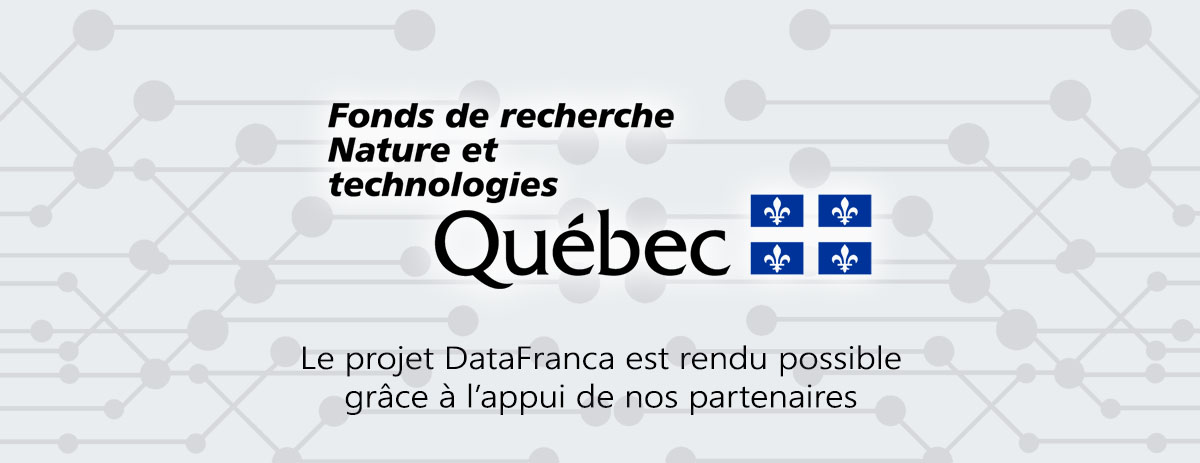
Contributeurs: Isaline Hodecent, wiki