« Évanescence du gradient » : différence entre les versions
m (Remplacement de texte — « Termes privilégiés » par « Français ») |
Aucun résumé des modifications Balises : mobile edit mobile web edit |
||
Ligne 3 : | Ligne 3 : | ||
[[Category:Vocabulary]] Vocabulary | [[Category:Vocabulary]] Vocabulary | ||
[[Catégorie:Apprentissage profond]] Apprentissage profond | [[Catégorie:Apprentissage profond]] Apprentissage profond | ||
[[Category:scotty]] | |||
== Définition == | == Définition == | ||
Ligne 13 : | Ligne 13 : | ||
=== le problème de la disparition du gradient === | === le problème de la disparition du gradient === | ||
== Anglais == | == Anglais == | ||
Ligne 22 : | Ligne 22 : | ||
The vanishing gradient problem arises in very deep Neural Networks, typically Recurrent Neural Networks, that use activation functions whose gradients tend to be small (in the range of 0 from 1). Because these small gradients are multiplied during backpropagation, they tend to “vanish” throughout the layers, preventing the network from learning long-range dependencies. Common ways to counter this problem is to use activation functions like ReLUs that do not suffer from small gradients, or use architectures like LSTMs that explicitly combat vanishing gradients. The opposite of this problem is called the exploding gradient problem. | The vanishing gradient problem arises in very deep Neural Networks, typically Recurrent Neural Networks, that use activation functions whose gradients tend to be small (in the range of 0 from 1). Because these small gradients are multiplied during backpropagation, they tend to “vanish” throughout the layers, preventing the network from learning long-range dependencies. Common ways to counter this problem is to use activation functions like ReLUs that do not suffer from small gradients, or use architectures like LSTMs that explicitly combat vanishing gradients. The opposite of this problem is called the exploding gradient problem. | ||
• On the difficulty of training recurrent neural networks | • On the difficulty of training recurrent neural networks | ||
<br> | |||
<br> | |||
<br> | |||
Référence : https://openclassrooms.com/courses/utilisez-des-modeles-supervises-non-lineaires/empilez-les-perceptrons | |||
<br> | |||
<br> | |||
<br> | |||
<br> |
Version du 18 janvier 2019 à 13:21
Domaine
Vocabulary Apprentissage profond
Définition
Français
le problème de la disparition du gradient
Anglais
Vanishing Gradient Problem
The vanishing gradient problem arises in very deep Neural Networks, typically Recurrent Neural Networks, that use activation functions whose gradients tend to be small (in the range of 0 from 1). Because these small gradients are multiplied during backpropagation, they tend to “vanish” throughout the layers, preventing the network from learning long-range dependencies. Common ways to counter this problem is to use activation functions like ReLUs that do not suffer from small gradients, or use architectures like LSTMs that explicitly combat vanishing gradients. The opposite of this problem is called the exploding gradient problem.
• On the difficulty of training recurrent neural networks
Référence : https://openclassrooms.com/courses/utilisez-des-modeles-supervises-non-lineaires/empilez-les-perceptrons
Contributeurs: Claude Coulombe, Jacques Barolet, Patrick Drouin, Pierre Labreche, wiki
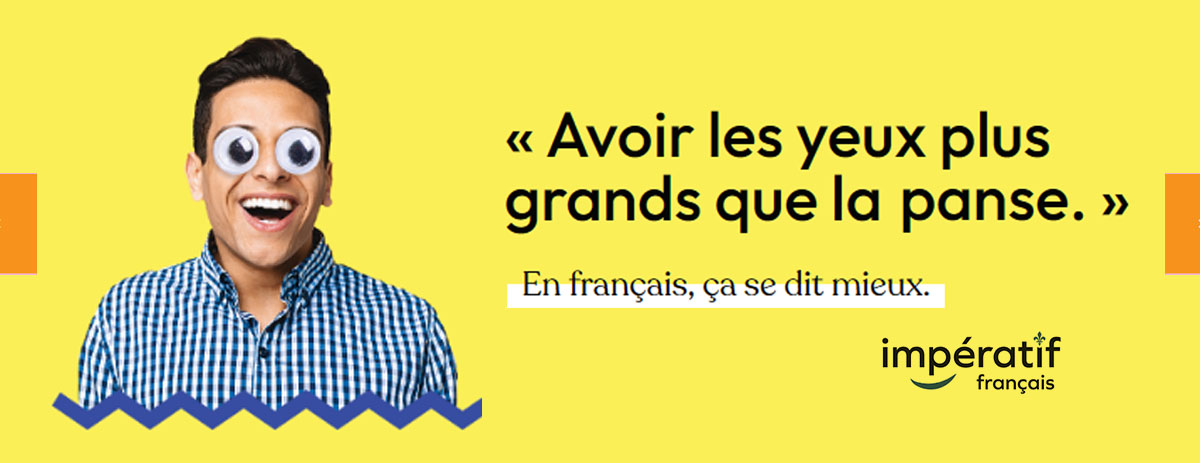