Méthode basée sur les graphes
en construction
Définition
XXXXXXXXX
Français
XXXXXXXXX
Anglais
Graph-based methods
Graph-based methods for semi-supervised learning use a graph representation of the data, with a node for each labeled and unlabeled example. The graph may be constructed using domain knowledge or similarity of examples; two common methods are to connect each data point to its {\displaystyle k}k nearest neighbors or to examples within some distance {\displaystyle \epsilon }\epsilon . The weight {\displaystyle W_{ij}}W_{ij} of an edge between {\displaystyle x_{i}}x_{i} and {\displaystyle x_{j}}x_{j} is then set to {\displaystyle e^{\frac {-\|x_{i}-x_{j}\|^{2}}{\epsilon }}}{\displaystyle e^{\frac {-\|x_{i}-x_{j}\|^{2}}{\epsilon }}}.
Within the framework of manifold regularization,[10][11] the graph serves as a proxy for the manifold. A term is added to the standard Tikhonov regularization problem to enforce smoothness of the solution relative to the manifold (in the intrinsic space of the problem) as well as relative to the ambient input space. The minimization problem becomes
Contributeurs: Imane Meziani, Patrick Drouin, wiki
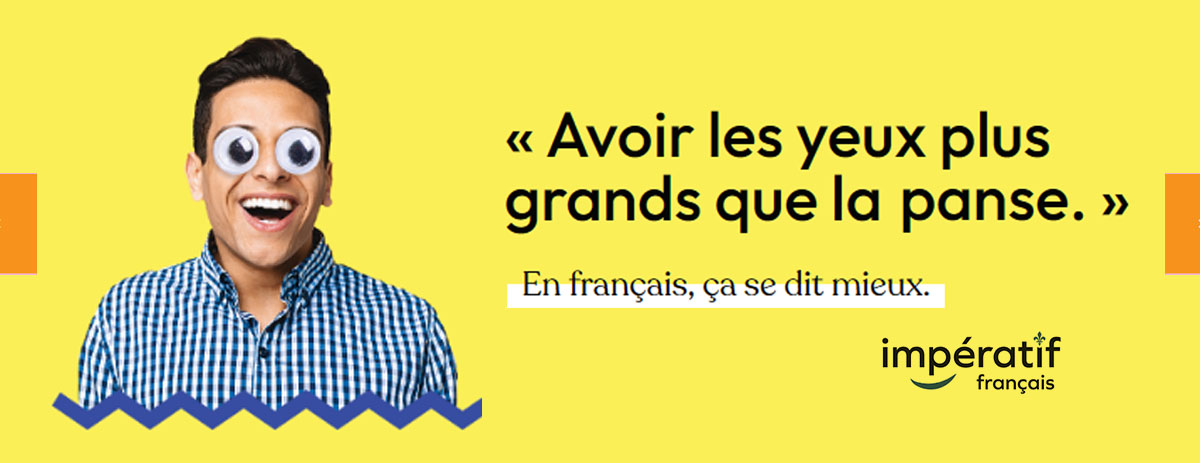