Données hors distribution
en construction
Définition
XXXXXXXXX
Français
XXXXXXXXX
Anglais
out-of-distribution data
A crucial criterion for deploying a strong classifier in many real-world machine learning applications is statistically or adversarially detecting test samples chosen sufficiently far away from the training distribution. Many classification tasks, such as speech recognition, object detection, and picture classification, have been done with great accuracy using neural networks (DNNs). However, determining the prediction uncertainty is still a difficult task. Predictive uncertainty that is well-calibrated is essential since it can be used in a variety of machine learning applications. Neural networks employing the softmax classifier, on the other hand, are known to yield significantly overconfident results. Loss is suitable for applications requiring tolerance such as product suggestions, it is risky to utilize those kinds of systems in intolerant fields such as robotics or medicine because they can result in fatal mishaps. When possible, an effective AI system should be able to generalize to OOD cases, flagging those beyond its capacity and requesting human intervention. hile in distribution examples are likely to have the same false patterns as OOD examples, neural network models can significantly rely on spurious cues and annotation artifacts inherent in the odd training data. Because the training data cannot cover all aspects of a distribution, the model’s capacity to generalize is limited
[XXXXXXX Source : XXX ]
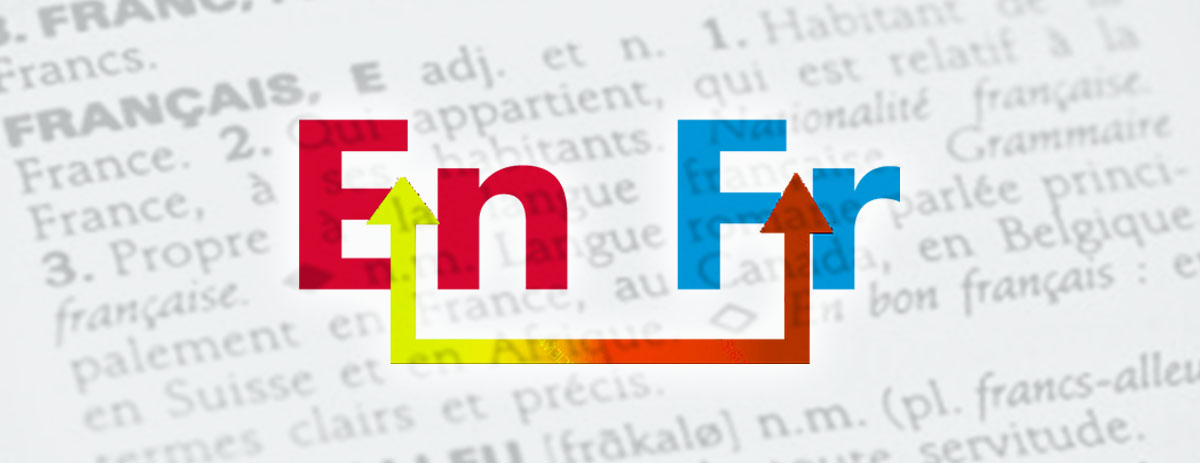
Contributeurs: Imane Meziani, wiki