Apprentissage antagoniste
en construction
Définition
XXXXXXXXX
Français
XXXXXXXXX
Anglais
Adversarial Machine Learning
Adversarial Machine Learning is a collection of techniques to train neural networks on how to spot intentionally misleading data or behaviors. This differs from the standard classification problem in machine learning, since the goal is not just to spot “bad” inputs, but preemptively locate vulnerabilities and craft more flexible learning algorithms.
While there are countless types of attacks and vectors to exploit machine learning systems, in broad strokes all attacks boil down to either:
Classification evasion: The most common form of attack, where the adversary seeks to hide malicious content to pass the algorithm’s filters. Data poisoning: This more sophisticated attack tries to manipulate the learning process by introducing fake or misleading data that compromises the algorithm’s outputs. Note: this field of training is security-oriented, and not the same as generative adversarial networks (GAN), which is an unsupervised machine learning technique that pits two neural networks against one another to speed up the learning process.
Contributeurs: Imane Meziani, Patrick Drouin, wiki
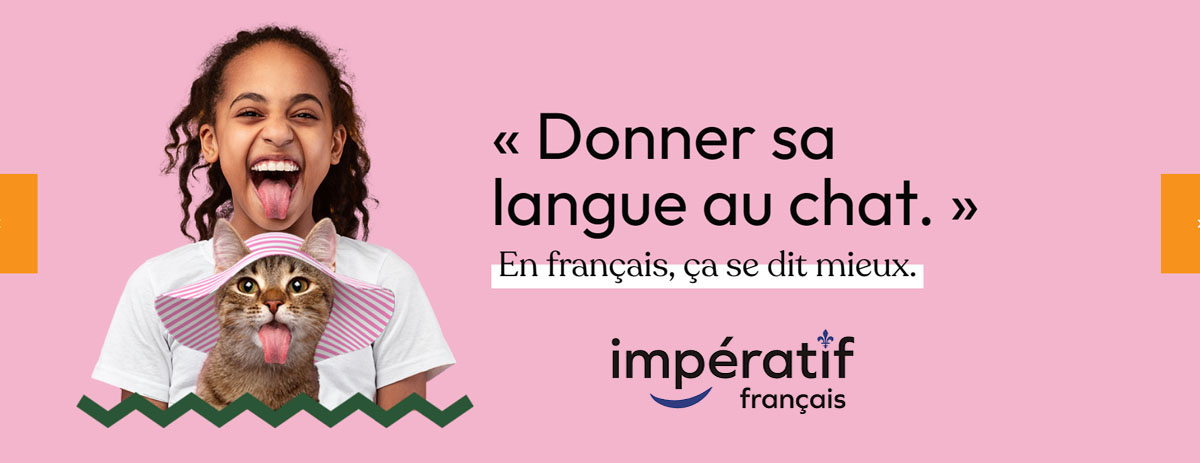