ACP éparse
en construction
Définition
XXXXXXXXX
Français
XXXXXXXXX
Anglais
Sparse PCA
Sparse principal component analysis (sparse PCA) is a specialised technique used in statistical analysis and, in particular, in the analysis of multivariate data sets. It extends the classic method of principal component analysis (PCA) for the reduction of dimensionality of data by introducing sparsity structures to the input variables.
A particular disadvantage of ordinary PCA is that the principal components are usually linear combinations of all input variables. Sparse PCA overcomes this disadvantage by finding linear combinations that contain just a few input variables.
Contemporary datasets often have the number of input variables ({\displaystyle p}p) comparable with or even much larger than the number of samples ({\displaystyle n}n). It has been shown that if {\displaystyle p/n}{\displaystyle p/n} does not converge to zero, the classical PCA is not consistent. But sparse PCA can retain consistency even if {\displaystyle p\gg n.}{\displaystyle p\gg n.}[1]
Contributeurs: Imane Meziani, wiki
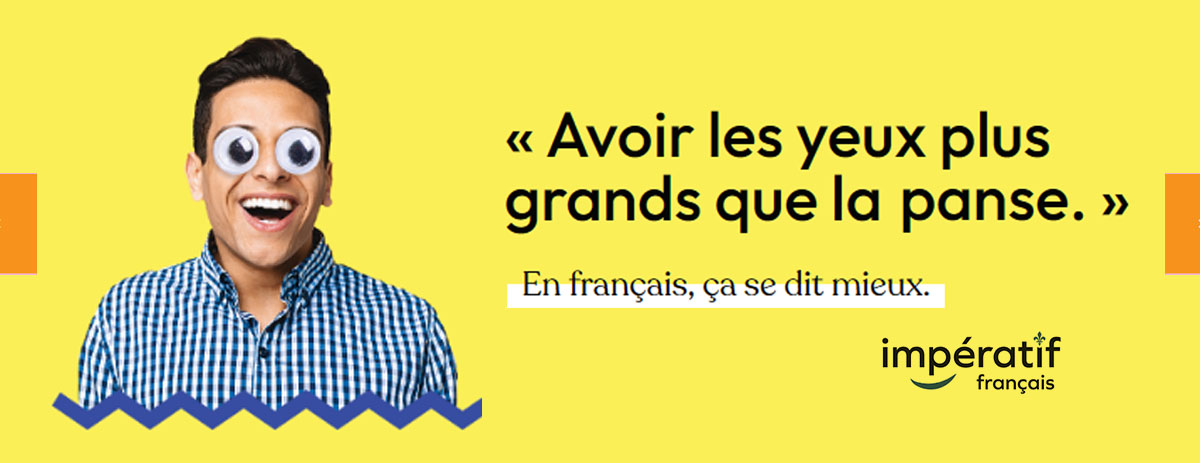