« Apprentissage automatique minuscule » : différence entre les versions
m (Imeziani a déplacé la page TinyML vers Apprentissage automatique minuscule) |
Aucun résumé des modifications |
||
Ligne 1 : | Ligne 1 : | ||
== Définition == | == Définition == | ||
Technique d'apprentissage automatique qui vise à permettre l'utilisation d'applications d'apprentissage automatique sur des dispositifs bon marché et limités en ressources et en énergie tels que des capteurs ou des microcontrôleurs dans le but d'effectuer des tâches automatisées. | |||
== Français == | == Français == | ||
''' | ''' AAMinuscule''' | ||
'''apprentissage automatique minuscule''' | |||
== Anglais == | == Anglais == | ||
''' TinyML''' | ''' TinyML''' | ||
'''tiny machine learning''' | |||
<small> | <small> | ||
[https://medium.com/starschema-blog/data-science-trends-to-rule-2022-68ab4c93cd2 Source : | [https://medium.com/starschema-blog/data-science-trends-to-rule-2022-68ab4c93cd2 Source : Medium] | ||
[https://towardsdatascience.com/an-introduction-to-tinyml-4617f314aa79 Source: Towards Data Science] | |||
[[Catégorie: | [[Catégorie:publication]] |
Version du 15 septembre 2022 à 10:55
Définition
Technique d'apprentissage automatique qui vise à permettre l'utilisation d'applications d'apprentissage automatique sur des dispositifs bon marché et limités en ressources et en énergie tels que des capteurs ou des microcontrôleurs dans le but d'effectuer des tâches automatisées.
Français
AAMinuscule
apprentissage automatique minuscule
Anglais
TinyML
tiny machine learning
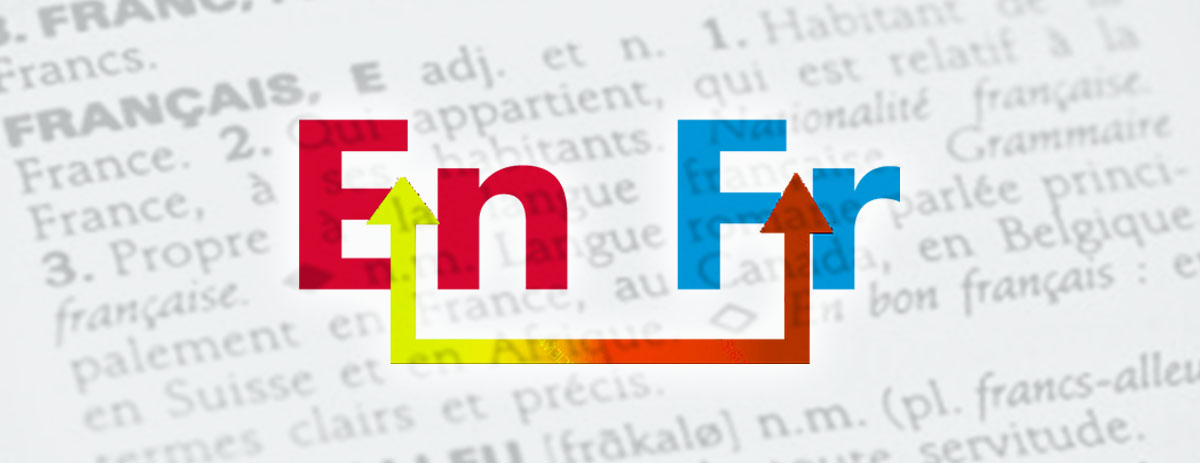
Contributeurs: Imane Meziani, wiki