« Apprentissage du classement » : différence entre les versions
(Page créée avec « ==en construction== == Définition == XXXXXXXXX == Français == ''' XXXXXXXXX ''' == Anglais == ''' Learning to rank''' Learning to rank[1] or machine-learned ranking... ») |
Aucun résumé des modifications |
||
Ligne 10 : | Ligne 10 : | ||
''' Learning to rank''' | ''' Learning to rank''' | ||
Learning to rank | Learning to rank or machine-learned ranking (MLR) is the application of machine learning, typically supervised, semi-supervised or reinforcement learning, in the construction of ranking models for information retrieval systems.[2] Training data consists of lists of items with some partial order specified between items in each list. This order is typically induced by giving a numerical or ordinal score or a binary judgment (e.g. "relevant" or "not relevant") for each item. The ranking model purposes to rank, i.e. producing a permutation of items in new, unseen lists in a similar way to rankings in the training data. | ||
<small> | <small> |
Version du 3 janvier 2022 à 17:10
en construction
Définition
XXXXXXXXX
Français
XXXXXXXXX
Anglais
Learning to rank
Learning to rank or machine-learned ranking (MLR) is the application of machine learning, typically supervised, semi-supervised or reinforcement learning, in the construction of ranking models for information retrieval systems.[2] Training data consists of lists of items with some partial order specified between items in each list. This order is typically induced by giving a numerical or ordinal score or a binary judgment (e.g. "relevant" or "not relevant") for each item. The ranking model purposes to rank, i.e. producing a permutation of items in new, unseen lists in a similar way to rankings in the training data.
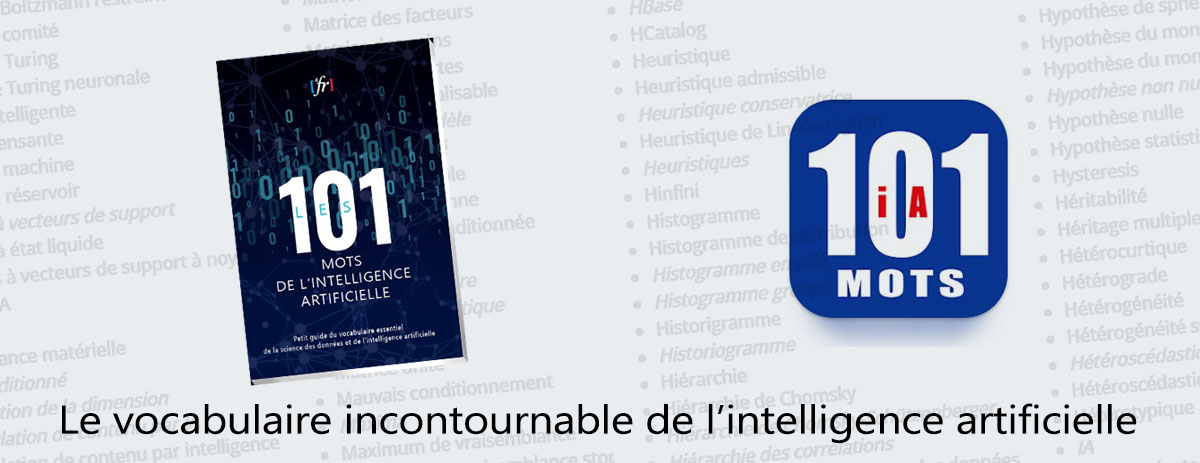
Contributeurs: Amanda Clément, wiki