« Cote logarithmique » : différence entre les versions
Aucun résumé des modifications Balise : Éditeur de wikicode 2017 |
Aucun résumé des modifications |
||
Ligne 1 : | Ligne 1 : | ||
== en construction == | == en construction == | ||
== Définition == | == Définition == | ||
Ligne 10 : | Ligne 8 : | ||
== Anglais == | == Anglais == | ||
'''intercept''' | '''intercept''' | ||
use of intercept in regression? : | |||
For linear models, the intercept is the value of the linear predictor when all covariates are zero. In linear regression, this is equivalent to the y-intercept of the line of best fit. In logistic regression, it is the log odds of the baseline group. Suppose we did not add an intercept term for the regression. We would then be positing that when all covariates are 0, the linear predictor is 0. In summation, we add the bias to improve interpretability and add flexibility to the model. | |||
Having no independent variables means no prediction can be made. Suppose you have a model for cancer incidence based on age. Since you do not know my age, you can not put my data into your model, and thus you can not make predictions. | |||
<small> | <small> | ||
[https://www.apprentissageprofond.org/ Source : ''L'apprentissage profond'', Ian Goodfellow, Yoshua Bengio et Aaron Courville Éd. Massot 2018 ] | [https://www.apprentissageprofond.org/ Source : ''L'apprentissage profond'', Ian Goodfellow, Yoshua Bengio et Aaron Courville Éd. Massot 2018 ] | ||
[https://stats.stackexchange.com/questions/380201/machine-learning-use-of-intercept-in-regression Source : stackexchange.com] | |||
[[Catégorie:vocabulaire]] | |||
[[Catégorie:App-profond-livre]] |
Version du 21 janvier 2021 à 08:47
en construction
Définition
Français
ordonnée à l'origine
Anglais
intercept
use of intercept in regression? : For linear models, the intercept is the value of the linear predictor when all covariates are zero. In linear regression, this is equivalent to the y-intercept of the line of best fit. In logistic regression, it is the log odds of the baseline group. Suppose we did not add an intercept term for the regression. We would then be positing that when all covariates are 0, the linear predictor is 0. In summation, we add the bias to improve interpretability and add flexibility to the model.
Having no independent variables means no prediction can be made. Suppose you have a model for cancer incidence based on age. Since you do not know my age, you can not put my data into your model, and thus you can not make predictions.
Source : L'apprentissage profond, Ian Goodfellow, Yoshua Bengio et Aaron Courville Éd. Massot 2018
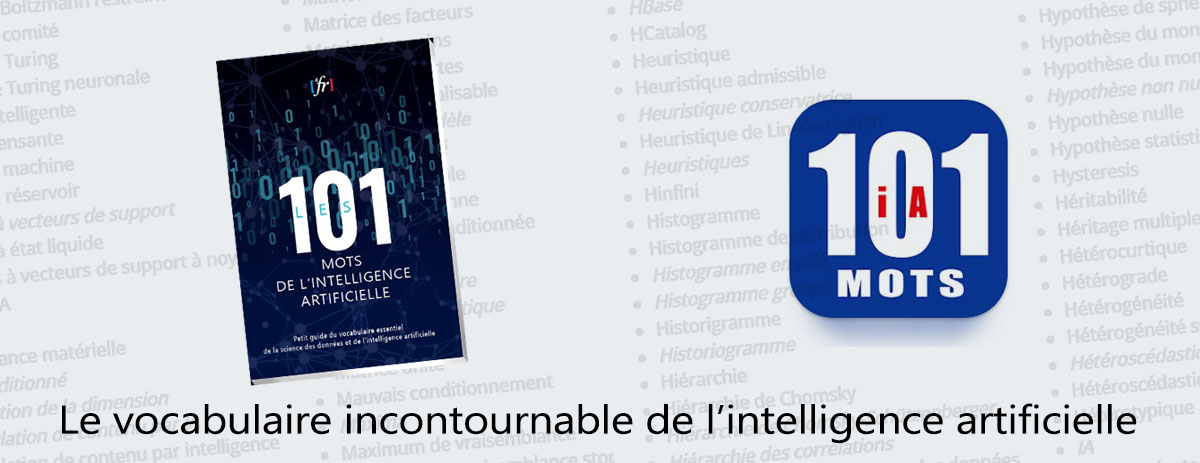
Contributeurs: Jacques Barolet, wiki