« Surface d'erreur » : différence entre les versions
Aucun résumé des modifications |
Aucun résumé des modifications |
||
Ligne 1 : | Ligne 1 : | ||
== en construction == | |||
[[Catégorie:Vocabulary]] | |||
[[Catégorie:Apprentissage automatique]] | |||
[[Catégorie:UNSW]] | |||
== Définition == | == Définition == | ||
XXXXXXXXXXXXXXX | |||
== Français == | == Français == | ||
''' | '''XXXXXXXXXXXXXXX ''' | ||
== Anglais == | == Anglais == | ||
'''error surface''' | '''error surface''' | ||
When total error of a backpropagation-trained neural network is expressed as a function of the weights, and graphed (to the extent that this is possible with a large number of weights), the result is a surface termed the error surface. The course of learning can be traced on the error surface: as learning is supposed to reduce error, when the learning algorithm causes the weights to change, the current point on the error surface should descend into a valley of the error surface. | |||
The "point" defined by the current set of weights is termed a point in weight space. Thus weight space is the set of all possible values of the weights. | |||
See also local minimum and gradient descent. | |||
<small> | <small> | ||
[http://www.cse.unsw.edu.au/~billw/dictionaries/mldict.html Source : INWS machine learning dictionary] ] | [http://www.cse.unsw.edu.au/~billw/dictionaries/mldict.html Source : INWS machine learning dictionary] ] | ||
Version du 24 octobre 2021 à 12:32
en construction
Définition
XXXXXXXXXXXXXXX
Français
XXXXXXXXXXXXXXX
Anglais
error surface
When total error of a backpropagation-trained neural network is expressed as a function of the weights, and graphed (to the extent that this is possible with a large number of weights), the result is a surface termed the error surface. The course of learning can be traced on the error surface: as learning is supposed to reduce error, when the learning algorithm causes the weights to change, the current point on the error surface should descend into a valley of the error surface.
The "point" defined by the current set of weights is termed a point in weight space. Thus weight space is the set of all possible values of the weights.
See also local minimum and gradient descent.
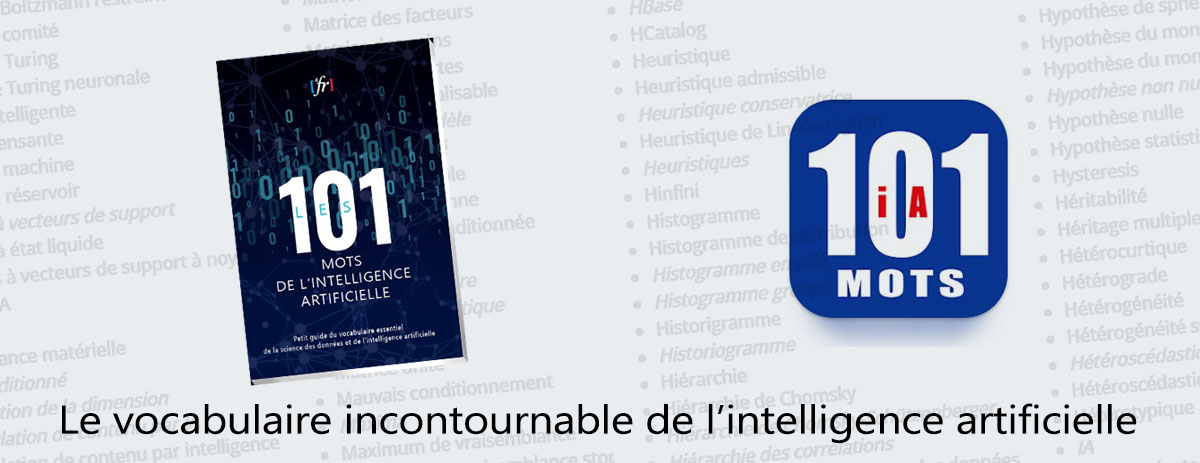
Contributeurs: Claire Gorjux, wiki