Valeur informationelle
en construction
Définition
XXXXXXXXX
Français
XXXXXXXXX
Anglais
Informativeness
The learning of mixture models can be viewed as a clustering problem. Indeed, given data samples independently generated from a mixture of distributions, we often would like to find the {\it correct target clustering} of the samples according to which component distribution they were generated from. For a clustering problem, practitioners often choose to use the simple k-means algorithm. k-means attempts to find an {\it optimal clustering} that minimizes the sum-of-squares distance between each point and its cluster center. In this paper, we consider fundamental (i.e., information-theoretic) limits of the solutions (clusterings) obtained by optimizing the sum-of-squares distance. In particular, we provide sufficient conditions for the closeness of any optimal clustering and the correct target clustering assuming that the data samples are generated from a mixture of spherical Gaussian distributions. We also generalize our results to log-concave distributions. Moreover, we show that under similar or even weaker conditions on the mixture model, any optimal clustering for the samples with reduced dimensionality is also close to the correct target clustering. These results provide intuition for the informativeness of k-means (with and without dimensionality reduction) as an algorithm for learning mixture models.
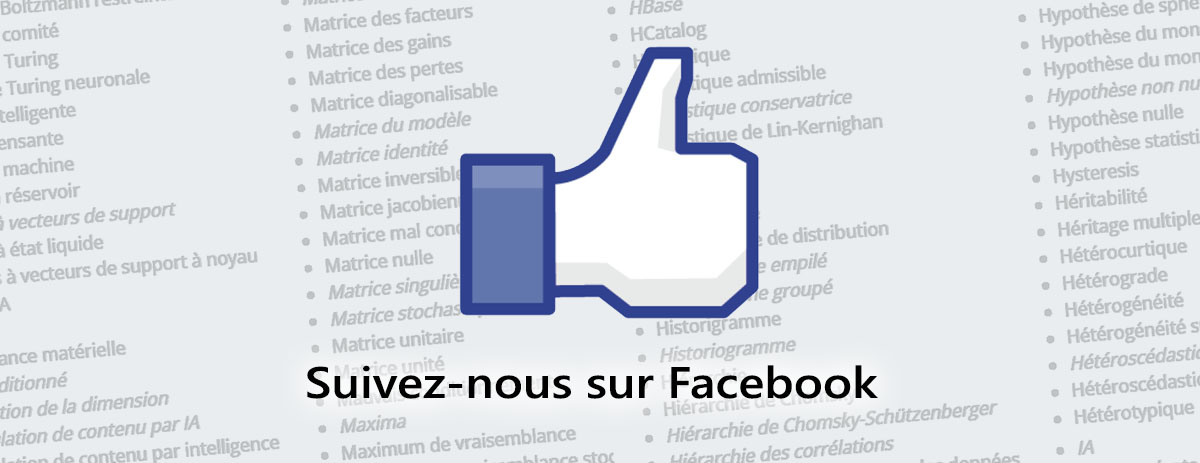
Contributeurs: Patrick Drouin, wiki