« Apprentissage par renforcement et rétroaction humaine » : différence entre les versions
Aucun résumé des modifications |
Aucun résumé des modifications |
||
(4 versions intermédiaires par 3 utilisateurs non affichées) | |||
Ligne 17 : | Ligne 17 : | ||
'''ARRH''' | '''ARRH''' | ||
''' apprentissage par renforcement basée sur la rétroaction humaine ''' | |||
'''ARBRH''' | |||
''' apprentissage par renforcement avec retour humain ''' | |||
== Anglais == | == Anglais == | ||
Ligne 30 : | Ligne 36 : | ||
--- | --- | ||
Learning from instructions and human feedback are thought to be at the core of recent advances in instruction following large language models (LLMs). While recent efforts such as Open Assistant, Vicuna, and Alpaca have advanced our understanding of instruction fine-tuning, the same cannot be said for RLHF-style algorithms that learn directly from human feedback. AlpacaFarm aims to address this gap by enabling fast, low-cost research and development on methods that learn from human feedback. We identify three main difficulties with studying RLHF-style algorithms: the high cost of human preference data, the lack of trustworthy evaluation, and the absence of reference implementations. --> | Learning from instructions and human feedback are thought to be at the core of recent advances in instruction following large language models (LLMs). While recent efforts such as Open Assistant, Vicuna, and Alpaca have advanced our understanding of instruction fine-tuning, the same cannot be said for RLHF-style algorithms that learn directly from human feedback. AlpacaFarm aims to address this gap by enabling fast, low-cost research and development on methods that learn from human feedback. We identify three main difficulties with studying RLHF-style algorithms: the high cost of human preference data, the lack of trustworthy evaluation, and the absence of reference implementations. --> | ||
==Sources== | ==Sources== | ||
Ligne 41 : | Ligne 46 : | ||
[https://www.journaldunet.com/solutions/dsi/1518637-chatgpt-l-intelligence-artificielle-peut-elle-tenir-ses-promesses/ Source : Journal du Net] | [https://www.journaldunet.com/solutions/dsi/1518637-chatgpt-l-intelligence-artificielle-peut-elle-tenir-ses-promesses/ Source : Journal du Net] | ||
[[Catégorie:Intelligence artificielle]] | |||
[[Catégorie:GRAND LEXIQUE FRANÇAIS]] | [[Catégorie:GRAND LEXIQUE FRANÇAIS]] | ||
[[Catégorie:101]] |
Dernière version du 3 octobre 2024 à 17:14
Définition
En apprentissage automatique, l'apprentissage par renforcement et rétroaction humaine (ARRH) est une technique d'amélioration des performances d'un agent à partir de rétroactions humaines.
On commence par entraîner un modèle de récompense sur des résultats annotés par des rétroactions humaines. Ensuite, on utilise ce modèle comme fonction de récompense pour améliorer la politique d'un agent à l'aide de l'apprentissage par renforcement grâce à un algorithme d'optimisation.
Compléments
Ce type d'apprentissage est utilisé dans les jeux AlphaGo et les générateurs de texte fondés sur les grands modèles de langues.
Le modèle de récompense est pré-entraîné pour que la politique soit optimisée afin de prédire si une sortie est bonne (récompense élevée) ou mauvaise (récompense faible ou pénalité).
Français
apprentissage par renforcement et rétroaction humaine
apprentissage par renforcement avec rétroaction humaine
apprentissage par renforcement à partir de la rétroaction humaine
ARRH
apprentissage par renforcement basée sur la rétroaction humaine
ARBRH
apprentissage par renforcement avec retour humain
Anglais
reinforcement learning from human feedback
RLHF
reinforcement learning from human preferences
Sources
Contributeurs: Arianne , Claude Coulombe, Patrick Drouin, wiki
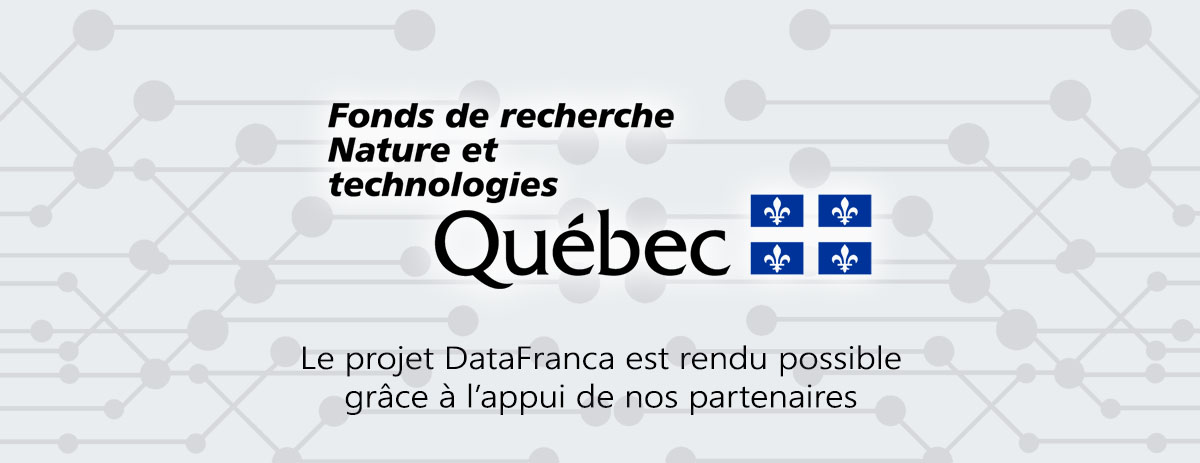