« Pondération attentionnelle » : différence entre les versions
(Page créée avec « == Définition == XXXXXXXXX == Français == ''' XXXXXXXXX ''' == Anglais == ''' Attention-Weighting''' Attention-weighting is a technique by which the model learns which part of the incoming sequence needs to be focused on. Think of it as the ‘Eye of Sauron’ scanning everything at all times and throwing light on the parts that are relevant. == Source == [https://arxiv.org/pdf/2211.07714 Source : arxiv] Catégorie:vocabulary ») |
Aucun résumé des modifications |
||
Ligne 1 : | Ligne 1 : | ||
== Définition == | == Définition == | ||
Technique qui permet au modèle d'apprendre si une certaine partie de la séquence d'entrée nécessite une attention particulière. | |||
Voir aussi '''[[Mécanisme d'attention]]''' | |||
== Français == | == Français == | ||
Ligne 7 : | Ligne 10 : | ||
== Anglais == | == Anglais == | ||
''' Attention-Weighting''' | ''' Attention-Weighting''' | ||
'''Attention Weights''' | |||
Attention-weighting is a technique by which the model learns which part of the incoming sequence needs to be focused on. Think of it as the ‘Eye of Sauron’ scanning everything at all times and throwing light on the parts that are relevant. | Attention-weighting is a technique by which the model learns which part of the incoming sequence needs to be focused on. Think of it as the ‘Eye of Sauron’ scanning everything at all times and throwing light on the parts that are relevant. | ||
In natural language processing, this usually means assigning different levels of importance to different words in a sentence. It assigns importance to each word by calculating "soft" weights for the word's numerical representation, known as its embedding, within a specific section of the sentence called the context window to determine its importance. The calculation of these weights can occur simultaneously in models called transformers, or one by one in models known as recurrent neural networks. Unlike "hard" weights, which are predetermined and fixed during training, "soft" weights can | |||
== Source == | == Source == | ||
[https://arxiv.org/pdf/2211.07714 Source : arxiv] | [https://arxiv.org/pdf/2211.07714 Source : arxiv] | ||
[https://en.wikipedia.org/wiki/Attention_(machine_learning) Source: wiki] | |||
[[Catégorie:vocabulary]] | [[Catégorie:vocabulary]] |
Version du 13 août 2024 à 16:33
Définition
Technique qui permet au modèle d'apprendre si une certaine partie de la séquence d'entrée nécessite une attention particulière.
Voir aussi Mécanisme d'attention
Français
XXXXXXXXX
Anglais
Attention-Weighting Attention Weights
Attention-weighting is a technique by which the model learns which part of the incoming sequence needs to be focused on. Think of it as the ‘Eye of Sauron’ scanning everything at all times and throwing light on the parts that are relevant.
In natural language processing, this usually means assigning different levels of importance to different words in a sentence. It assigns importance to each word by calculating "soft" weights for the word's numerical representation, known as its embedding, within a specific section of the sentence called the context window to determine its importance. The calculation of these weights can occur simultaneously in models called transformers, or one by one in models known as recurrent neural networks. Unlike "hard" weights, which are predetermined and fixed during training, "soft" weights can
Source
Contributeurs: Arianne , Patrick Drouin, wiki
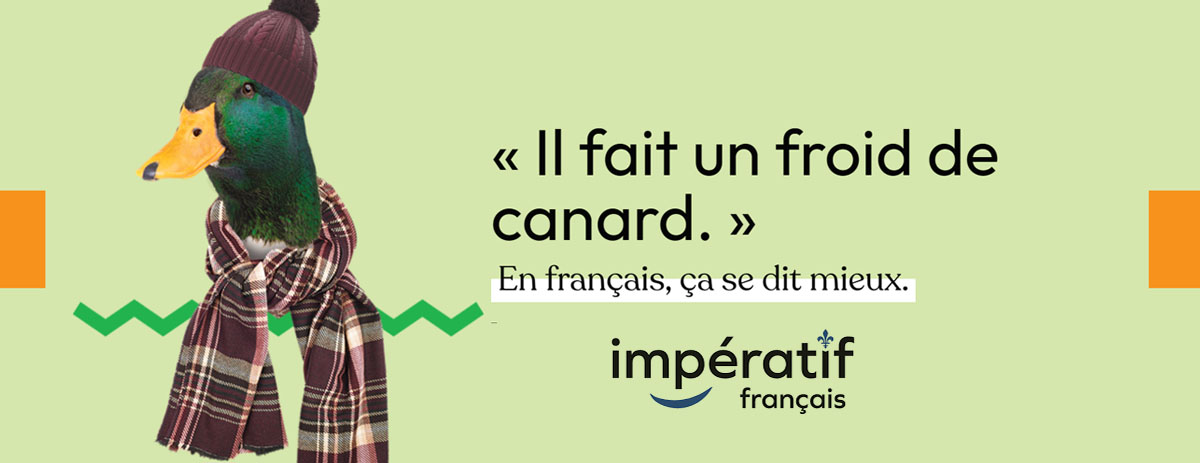